- Advanced Techniques in Economic Forecasting: Mastering the Dynamics of Economics Models
- Unveiling the Core Principles and Frameworks for Precision Economic Projections
- Advanced Applications of DSGE Models in Economic Forecasting
- Exploring the Intricacies of Econometrics Dynamics through Innovative Modeling Techniques
- Advanced Techniques and Innovations in DSGE Modeling for Enhanced Economic Forecasting
- Policy Implications of Advanced Economic Models: Guiding Decision-Making in Uncertain Times
- Conclusion:
In the realm of advanced economic forecasting, Dynamic Stochastic General Equilibrium (DSGE) models stand as a sophisticated tool for analyzing complex macroeconomic phenomena. As an expert guiding students through their econometrics assignments, I emphasize the pivotal role DSGE models play in understanding the intricacies of economic systems. These models integrate dynamic elements, uncertainty, and shocks into a unified framework, allowing for a comprehensive examination of economic dynamics. If you need help with your econometrics assignment, understanding DSGE models and their application is essential for tackling advanced economic forecasting tasks effectively.
DSGE models employ rigorous mathematical techniques to capture the interplay of various economic variables over time, considering how shocks and uncertainties propagate through the system. Guiding students in their econometrics assignments involves unraveling the complexities of model specification, estimation, and interpretation. DSGE models demand a solid grasp of economic theory, statistical methods, and computational skills, making them an intellectually challenging yet rewarding area of study.
Furthermore, students learn to address policy questions, evaluate the impact of shocks, and conduct scenario analyses using DSGE models. The expert guidance involves honing students' skills in parameter estimation, model calibration, and assessing the model's goodness of fit. By delving into DSGE models, students not only enhance their econometric proficiency but also gain valuable insights into macroeconomic dynamics, contributing to their ability to make informed predictions and policy recommendations in the ever-evolving economic landscape.
Advanced Techniques in Economic Forecasting: Mastering the Dynamics of Economics Models
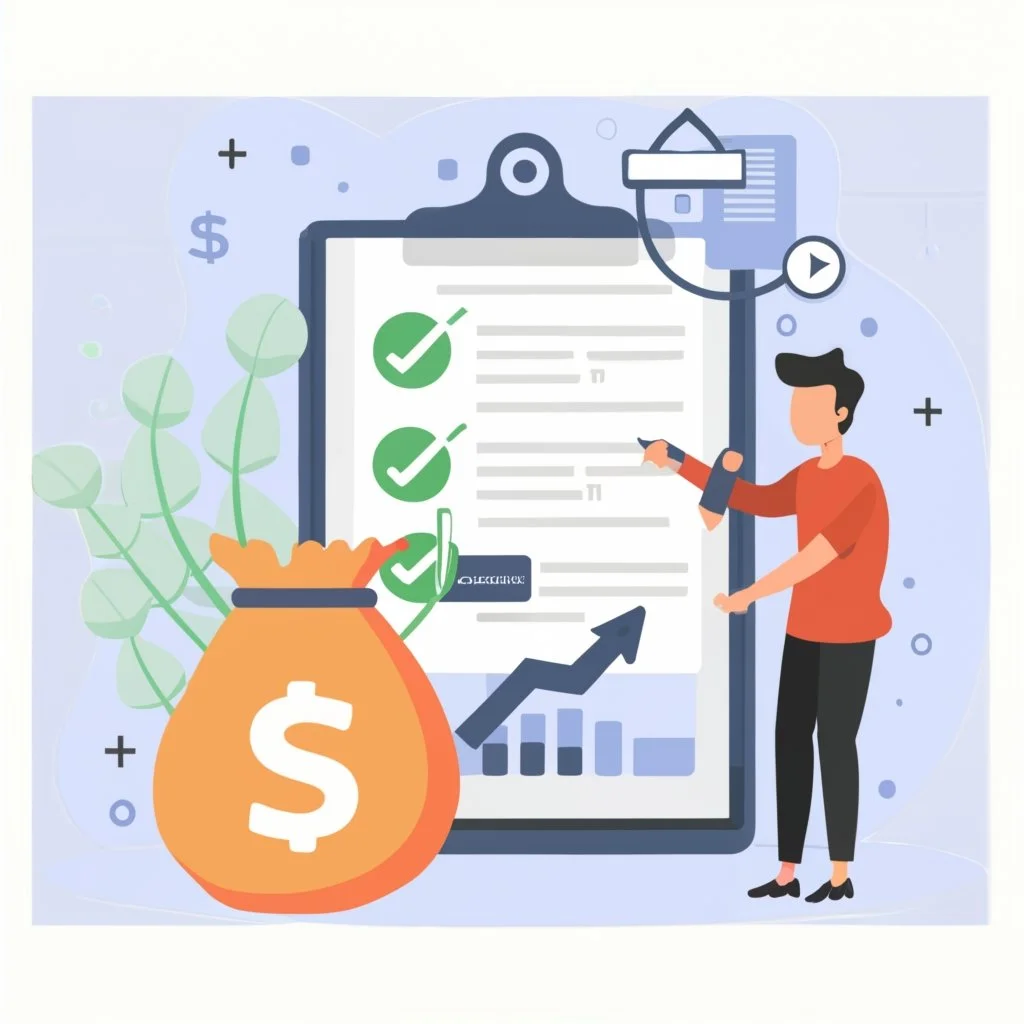
In the ever-evolving landscape of economic dynamics, mastering advanced techniques in economic forecasting is crucial for both scholars and practitioners. Economic forecasting, a cornerstone of econometrics, plays a pivotal role in decision-making processes for governments, businesses, and individuals alike. As an expert guiding students through the intricate world of econometrics assignments, I find it imperative to emphasize the significance of mastering advanced techniques to unlock the true potential of economic models.
Econometrics assignments often require students to grapple with intricate methodologies and statistical tools that form the backbone of economic forecasting. A fundamental understanding of basic econometric principles is essential, but to truly excel, students must delve into the realm of advanced techniques. These techniques not only enhance the accuracy of forecasts but also empower students to navigate the complexities inherent in economic systems.
One of the cornerstones of advanced economic forecasting is time series analysis. Time series data, representing observations over time, are ubiquitous in economic studies. Mastering techniques such as autoregressive integrated moving average (ARIMA) models and seasonal decomposition of time series (STL) allows students to capture and analyze temporal patterns, thereby improving the accuracy of economic predictions. Through meticulous guidance, students can comprehend the intricacies of identifying trends, seasonality, and the impact of external factors on time series data.
Furthermore, embracing machine learning (ML) techniques is paramount in the modern landscape of economic forecasting. The advent of big data has transformed the field, enabling economists to harness the predictive power of algorithms. As an expert guiding students, introducing them to supervised learning models like regression analysis, decision trees, and neural networks fosters a deeper understanding of how to leverage vast datasets for robust economic predictions. The integration of ML techniques not only refines forecasting accuracy but also equips students with valuable skills applicable in the broader data-driven landscape.
Stochastic processes, another advanced technique, are integral to understanding the inherent uncertainty in economic systems. By delving into the intricacies of stochastic models, students can grasp the probabilistic nature of economic variables and incorporate uncertainty into their forecasts. This not only enhances the robustness of predictions but also instills a sense of realism in economic modeling, acknowledging the dynamic and unpredictable nature of economic phenomena.
Cointegration and error correction models stand as pillars in the arsenal of advanced econometric techniques. These tools are particularly relevant when analyzing the long-term relationships between economic variables. By guiding students through the intricacies of cointegration, I empower them to uncover equilibrium relationships and understand the forces that drive the adjustment process towards long-term economic equilibrium. Such insights are invaluable in forecasting scenarios where understanding the dynamics of interrelated variables is paramount.
The mastery of advanced techniques in economic forecasting is a transformative journey for students navigating the complex realm of econometrics assignments. As an expert guiding them through this journey, my role extends beyond imparting theoretical knowledge to instilling practical skills that empower them to unravel the intricacies of economic models. Time series analysis, machine learning, stochastic processes, cointegration, and error correction models collectively form a toolkit that equips students to navigate the dynamic and uncertain landscape of economic forecasting, contributing not only to academic success but also preparing them for the challenges of real-world economic analysis.
Unveiling the Core Principles and Frameworks for Precision Economic Projections
In the realm of economic forecasting, precision is paramount, and unraveling the core principles and frameworks for precise economic projections is a task of utmost significance. As an expert guiding students through the intricate landscape of econometrics assignments, it is imperative to instill a deep understanding of foundational principles. At the nucleus of precision economic projections lies a judicious application of statistical tools and mathematical models.
Emphasizing the role of robust data collection and analysis, students are encouraged to comprehend the intricate relationships between economic variables. The precision in economic projections is fostered through the meticulous calibration of econometric models, ensuring they encapsulate the dynamic interplay of various factors influencing economic trends.
Furthermore, students are introduced to the significance of time-series analysis and regression techniques, pivotal components in crafting precise economic forecasts. The expert guidance extends to elucidating the intricacies of forecasting models, such as autoregressive integrated moving average (ARIMA) and vector autoregression (VAR), unveiling their application in different economic scenarios.
By equipping students with a comprehensive understanding of these core principles and frameworks, the expert ensures a solid foundation for mastering the art of precision economic projections. Nurturing analytical skills and fostering a keen insight into economic data, this guidance empowers students to navigate the complexities of econometrics assignments with confidence and proficiency. In essence, the expert's role extends beyond imparting knowledge; it cultivates a proficiency that empowers students to contribute meaningfully to the precision of economic projections in the broader professional landscape.
Advanced Applications of DSGE Models in Economic Forecasting
As an expert in the field of econometrics, guiding and assisting students in navigating the intricate world of economic modeling and forecasting is both a challenging and rewarding task. Among the various models that students encounter, Dynamic Stochastic General Equilibrium (DSGE) models stand out as powerful tools for analyzing complex economic systems and making predictions about future economic conditions. In this comprehensive guide, we will delve into the advanced applications of DSGE models in economic forecasting, shedding light on their intricacies and showcasing their relevance in shaping modern economic analysis.
Dynamic Stochastic General Equilibrium models represent a state-of-the-art approach to understanding economic fluctuations and forecasting future economic trends. Unlike simpler econometric models, DSGE models capture the dynamics of economic variables over time by incorporating the interplay of various factors such as technology shocks, fiscal policies, and monetary policies. These models offer a comprehensive framework for analyzing the impact of different shocks on the economy, making them indispensable in both academic research and real-world policy analysis.
One key feature of DSGE models is their ability to incorporate expectations and rational behavior. This sets them apart from traditional models that assume agents have perfect foresight or rely on backward-looking expectations. In a DSGE framework, agents form expectations based on all available information, including past and present data. This realistic portrayal of expectations allows for a more nuanced understanding of how economic agents respond to changes in the economic environment, providing a valuable tool for forecasting.
Econometrics students engaging with DSGE models often grapple with the technical aspects of model calibration and estimation. These models require a deep understanding of economic theory, statistical techniques, and computational methods. Calibration involves setting the model parameters to match certain moments in the data, while estimation involves using statistical methods to infer the unknown parameters. Advanced students are encouraged to explore the nuances of Bayesian estimation techniques, which allow for a more flexible and robust approach to parameter estimation.
One of the notable applications of DSGE models is in monetary policy analysis. Central banks around the world utilize these models to assess the impact of interest rate changes, inflation targeting, and other policy measures on key economic variables. For econometrics students aspiring to work in central banks or policy research institutions, a solid grasp of DSGE modeling is essential. Moreover, understanding how to interpret the model's results and communicate them effectively to policymakers is a crucial skill that sets successful economists apart.
Another frontier in DSGE modeling is the incorporation of financial frictions and heterogeneous agents. Advanced DSGE models go beyond the traditional assumption of a representative agent and account for differences in wealth, risk aversion, and access to credit. This allows for a more realistic representation of the economy and a better understanding of the transmission mechanisms of various shocks. Econometrics students exploring these advanced applications gain valuable insights into the complexities of the financial system and its interactions with the broader economy.
The advanced applications of DSGE models in economic forecasting offer a rich and challenging terrain for econometrics students. As an expert in the field, guiding students through the intricacies of model calibration, estimation, and interpretation is a gratifying endeavor. DSGE models not only provide a powerful analytical framework for understanding economic dynamics but also equip students with the skills and knowledge necessary to navigate the evolving landscape of economic research and policymaking. Embracing the complexities of DSGE modeling ensures that future economists are well-equipped to contribute meaningfully to the field and make informed predictions about the ever-changing economic landscape.
Exploring the Intricacies of Econometrics Dynamics through Innovative Modeling Techniques
Delving into the realm of econometrics dynamics unveils a multifaceted landscape where traditional models meet the demands of contemporary challenges. As an expert guiding students through their econometrics assignments, the exploration of intricacies becomes paramount. Innovative modeling techniques serve as the compass in navigating this complex terrain, empowering students to grasp the dynamic interplay of economic variables.
In this intellectual journey, we traverse beyond conventional linear regression models, embracing cutting-edge methodologies like time-series analysis, panel data models, and structural equation modeling. The dynamic nature of economic systems demands a nuanced approach, prompting the integration of machine learning algorithms and Bayesian inference into econometric frameworks. These techniques allow students to unravel hidden patterns, capture non-linear relationships, and address endogeneity concerns with finesse.
The econometrician's toolkit extends beyond statistical prowess to include a profound understanding of economic theory and domain-specific knowledge. Guiding students through this integration fosters a holistic comprehension of econometrics dynamics. Encouraging critical thinking and the application of these innovative models to real-world scenarios empowers students to contribute meaningfully to economic research and policy discussions.
As an expert mentor, navigating the intricacies of econometrics dynamics involves instilling a passion for innovation and a keen appreciation for the evolving landscape of economic analysis. Through adept guidance, students emerge equipped not only with technical proficiency but also with the ability to address the contemporary challenges that define the dynamic field of econometrics.
Advanced Techniques and Innovations in DSGE Modeling for Enhanced Economic Forecasting
In recent years, the landscape of economic forecasting has witnessed significant transformations, with advanced techniques and innovations pushing the boundaries of traditional modeling approaches. In this discourse, we will delve into the realm of DSGE modeling, exploring the cutting-edge methods that promise enhanced accuracy and reliability in economic forecasting.
Dynamic Stochastic General Equilibrium models serve as a cornerstone in understanding the complex interactions within an economy. Traditional DSGE models have evolved substantially, incorporating new methodologies and embracing technological advancements to address the inherent challenges of forecasting in dynamic economic environments.
One of the notable advancements in DSGE modeling is the incorporation of machine learning techniques. Machine learning algorithms, such as neural networks, have demonstrated remarkable capabilities in capturing non-linear relationships and patterns within economic data. By integrating these techniques into DSGE models, students can enhance their predictive accuracy, particularly in scenarios where traditional linear models fall short.
Furthermore, the adoption of Bayesian methods has revolutionized parameter estimation in DSGE models. Bayesian techniques allow for the incorporation of prior information and the continuous updating of estimates as new data becomes available. This not only improves the precision of parameter estimates but also enhances the model's adaptability to changing economic conditions, providing students with a more robust framework for their econometric assignments.
Another key innovation lies in the development of heterogeneous agent models within the DSGE framework. Unlike traditional models that assume homogeneity among agents, heterogeneous agent models recognize diverse behaviors and decision-making processes among individuals or firms. This innovation allows for a more nuanced representation of economic agents, better reflecting the complexities of real-world economic systems.
In the context of economic forecasting, it is crucial to account for uncertainties and external shocks. The integration of scenario analysis and stress testing techniques in DSGE models enables students to explore the impact of various economic scenarios on their forecasts. This not only enhances the models' predictive power but also equips students with the ability to assess the resilience of economic systems under different conditions.
Moreover, the advent of high-frequency data has opened new avenues for refining DSGE models. Real-time data feeds, such as those from financial markets and social media, can be integrated to improve the models' responsiveness to rapid changes in economic conditions. This real-time data integration empowers students to develop econometric assignments that reflect the most up-to-date information, providing a more accurate depiction of the economic landscape.
The field of DSGE modeling is undergoing a transformative phase with the integration of advanced techniques and innovations. As an expert guiding students in their econometrics assignments, it is essential to emphasize the importance of staying abreast of these developments. By embracing machine learning, Bayesian methods, heterogeneous agent models, scenario analysis, and real-time data, students can elevate their DSGE models to new heights, unlocking the full potential of economic forecasting in an ever-changing world. Through continuous learning and adaptation, econometrics students can position themselves at the forefront of this exciting evolution in economic modeling.
Policy Implications of Advanced Economic Models: Guiding Decision-Making in Uncertain Times
In the ever-evolving landscape of economic policy, the advent of advanced economic models has significantly altered the dynamics of decision-making processes. As an expert guiding students through the intricacies of econometrics assignments, it becomes imperative to explore the policy implications of these sophisticated models, particularly in the context of navigating uncertainty in contemporary times.
Advanced economic models, often rooted in cutting-edge econometric techniques, play a pivotal role in shaping policy decisions. Policymakers, facing the challenges of a rapidly changing global economy, rely on these models to provide insights, forecast trends, and formulate strategies that can withstand the complexities of uncertain environments. The implications of these models extend far beyond the classroom, as they serve as powerful tools for shaping the economic landscape.
One notable policy implication of advanced economic models is the ability to enhance the precision and accuracy of economic forecasts. Econometric techniques such as time-series analysis, panel data methods, and machine learning algorithms enable economists to harness vast datasets and extract meaningful patterns. This, in turn, empowers policymakers to make more informed decisions based on a nuanced understanding of economic trends and potential future scenarios.
Furthermore, these models offer the capacity to simulate the impact of various policy interventions. By incorporating counterfactual analysis and scenario planning, policymakers can assess the potential outcomes of different policy choices before implementation. This proactive approach allows for the identification of optimal strategies and mitigates the risk of unintended consequences, a crucial consideration in uncertain times.
In the realm of fiscal and monetary policy, advanced economic models provide a nuanced understanding of the intricate relationships between variables. For instance, dynamic stochastic general equilibrium (DSGE) models allow economists to capture the interplay of various economic agents and shocks, providing a comprehensive framework for assessing the impact of policy changes. This insight is invaluable for policymakers seeking to fine-tune macroeconomic policies to achieve desired outcomes in the face of uncertainty.
Moreover, the integration of behavioral economics into advanced models adds a layer of realism by considering the psychological factors influencing economic decisions. This nuanced understanding of human behavior enhances the predictive power of economic models and enables policymakers to design interventions that resonate with real-world dynamics.
However, it is crucial to acknowledge the limitations and challenges associated with advanced economic models. The assumptions underlying these models, though necessary for tractability, may oversimplify the complexities of the real world. As an expert guiding students, fostering a critical mindset is essential, encouraging them to question assumptions and understand the trade-offs involved in model selection.
The policy implications of advanced economic models are profound, reshaping the landscape of decision-making in uncertain times. As an expert guiding students through econometrics assignments, the emphasis should be on cultivating a holistic understanding of these models, acknowledging their strengths and limitations. Equipping the next generation of economists with the skills to navigate the intricacies of advanced economic models ensures that they contribute meaningfully to the formulation of robust policies that stand the test of uncertainty in the ever-evolving global economic arena.
Conclusion:
In conclusion, Dynamic Stochastic General Equilibrium (DSGE) models represent a sophisticated and powerful tool in the realm of advanced economic forecasting techniques. As an expert guiding students through their econometrics assignments, it is evident that the incorporation of DSGE models introduces a dynamic and stochastic dimension, allowing for a more nuanced understanding of economic phenomena.
These models go beyond traditional static frameworks by incorporating time dynamics and stochastic elements, reflecting the inherent uncertainty and fluctuations present in real-world economies. The emphasis on microeconomic foundations and the explicit consideration of agents' behavior enhance the realism of DSGE models, providing a solid foundation for comprehensive economic analyses.
However, it is crucial to acknowledge the challenges associated with DSGE modeling. The complexity of these models demands a robust understanding of economic theory, advanced mathematical techniques, and computational skills. As such, guiding students through the intricacies of DSGE models requires a balanced approach, combining theoretical knowledge with practical application.
DSGE models have proven invaluable in policy analysis, enabling a deeper examination of the effects of various shocks and policy interventions on economic variables. The incorporation of expectations, forward-looking behavior, and the interplay between different sectors of the economy adds a layer of sophistication that contributes to more accurate and insightful economic forecasts.
Nonetheless, it is essential to instill a critical mindset in students, encouraging them to question assumptions, assess model limitations, and consider alternative specifications. As an expert mentor, fostering a thorough understanding of the strengths and weaknesses of DSGE models ensures that students are well-equipped to navigate the ever-evolving landscape of economic forecasting.
Dynamic Stochastic General Equilibrium models stand as a testament to the continuous evolution of economic modeling techniques. Guiding students through the intricacies of DSGE models not only equips them with advanced analytical skills but also instills a deeper appreciation for the complexities inherent in economic systems. Embracing the dynamic nature of DSGE models contributes to the development of more robust and insightful economic forecasts, preparing the next generation of economists for the challenges and opportunities that lie ahead.