- Unraveling Market Dynamics: A Primer on Financial Markets Econometrics
- Efficiency Metrics and Methodologies: A Comprehensive Econometric Approach
- Analyzing Market Efficiency through Econometric Models
- Risk Assessment in Financial Markets: A Quantitative Econometric Perspective
- Challenges in Quantitative Risk Assessment:
- Quantifying Uncertainty: Econometric Tools for Financial Risk Analysis
- Temporal Trends and Patterns: Harnessing Time Series Models for Financial Markets
- Conclusion:
As an expert guiding students through the intricate realm of econometrics in financial markets, it is paramount to emphasize the multifaceted nature of analyzing market efficiency and risk. Econometrics serves as the indispensable tool kit, allowing us to unravel complex relationships within financial data, providing insights that are crucial for decision-making. Whether you need help with your Econometrics assignment or simply seek to deepen your understanding of financial market analysis, mastering econometric techniques is essential for navigating the complexities of financial markets with confidence and precision.
Efficient market hypothesis (EMH) is a cornerstone, asserting that asset prices reflect all available information. Unraveling its nuances through econometric techniques, students explore the implications of information asymmetry and market efficiency on investment strategies. Time-series analysis, including autoregressive models, helps unveil patterns and trends, aiding in the identification of potential opportunities and risks.
Risk assessment, an integral facet, involves not only measuring volatility but also understanding the interplay of various factors influencing financial markets. From GARCH models to value-at-risk calculations, students delve into advanced econometric methodologies to quantify and manage risk effectively.
The econometric journey in financial markets encompasses model validation, addressing endogeneity concerns, and navigating the challenges of non-stationary data. Through hands-on assignments, students develop a profound understanding of econometric tools, enabling them to contribute meaningfully to the evolving landscape of financial analysis. As an expert guide, I facilitate their mastery of econometrics, fostering a deeper comprehension of the intricate dynamics shaping financial markets.
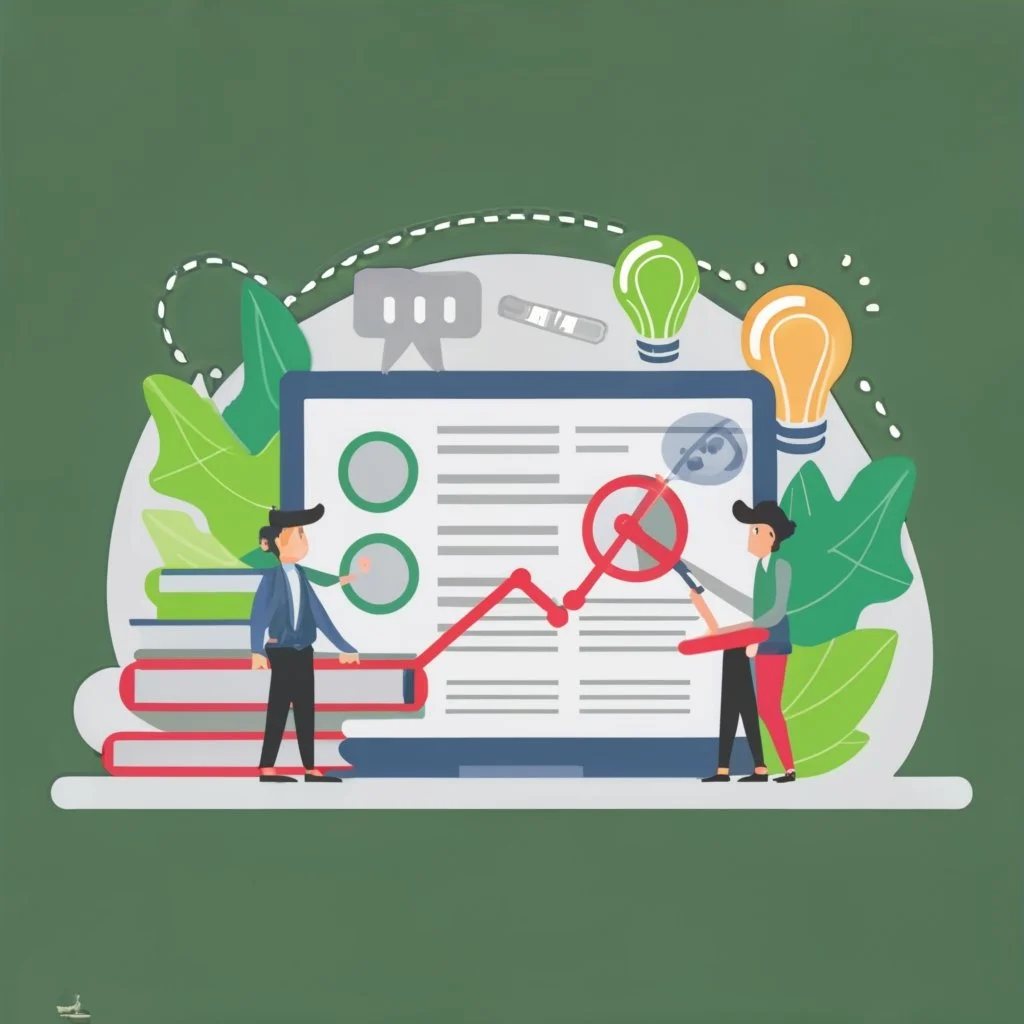
Unraveling Market Dynamics: A Primer on Financial Markets Econometrics
In the ever-evolving landscape of financial markets, understanding the intricacies of market dynamics is essential for both seasoned professionals and aspiring economists. The field of econometrics plays a pivotal role in unraveling the complexities of financial markets, offering a powerful toolkit to analyze and interpret economic and financial data. As an expert guiding students through their econometrics assignments, let's delve into the foundations and applications of econometrics in the context of financial markets.
Econometrics, the amalgamation of economic theory, mathematical statistics, and real-world data analysis, serves as a bridge between economic principles and empirical observations. In the realm of financial markets, econometrics provides a structured framework for modeling and analyzing various market dynamics, ranging from price movements to the intricate relationships between financial variables.
One of the fundamental applications of econometrics in financial markets is the modeling of asset prices. Students engaging with econometrics assignments often grapple with understanding the factors influencing stock prices, bond yields, or commodity values. Econometric techniques, such as time-series analysis and regression models, empower students to quantify and evaluate the impact of diverse variables on asset prices. Whether it's interest rates, inflation, or macroeconomic indicators, econometrics equips students with the tools to dissect the driving forces behind market fluctuations.
Volatility is another critical facet of financial markets that students frequently encounter in their assignments. The ability to measure and predict volatility is paramount for risk management and investment decision-making. Econometric models like GARCH (Generalized Autoregressive Conditional Heteroskedasticity) provide students with a robust framework to capture and analyze volatility patterns, allowing them to make informed predictions about future market movements.
Correlation and causality are essential concepts in financial markets, and econometrics provides the means to disentangle complex relationships between different variables. Students often grapple with determining whether a specific economic indicator causes a change in asset prices or if the observed correlation is merely coincidental. Through tools like Granger causality tests and panel data analysis, econometrics empowers students to discern causal relationships, enhancing their ability to make accurate predictions and informed investment decisions.
The efficiency of financial markets is a perennial topic in both academic and practical contexts. The Efficient Market Hypothesis (EMH) posits that asset prices reflect all available information, leaving no room for consistently outperforming the market. Econometrics enables students to test the validity of the EMH by evaluating the efficiency of market prices through statistical methods. Monte Carlo simulations, event studies, and cointegration analysis are some econometric tools that guide students in assessing the efficiency of financial markets and identifying potential anomalies.
As an expert mentor, it is crucial to emphasize the practical application of econometrics in addressing real-world financial challenges. Students should be encouraged to apply their econometric skills to analyze financial data sets, interpret results, and draw meaningful conclusions. Providing hands-on experience with industry-standard software like R, Python, or STATA enhances students' ability to translate theoretical knowledge into actionable insights.
The field of financial markets econometrics is a dynamic and indispensable discipline for students aspiring to comprehend the intricacies of market behavior. As an expert guiding students through their econometrics assignments, the emphasis should be on equipping them with the analytical tools necessary to unravel market dynamics, make informed predictions, and contribute meaningfully to the ever-evolving world of finance. Through a combination of theoretical understanding and practical application, students can develop the expertise needed to navigate the complex terrain of financial markets with confidence and proficiency.
Efficiency Metrics and Methodologies: A Comprehensive Econometric Approach
In the intricate realm of econometrics, understanding and implementing efficiency metrics and methodologies is paramount for students aiming to master the subject. As an expert guiding students through their econometrics assignments, I emphasize the importance of a comprehensive approach to efficiency measurement that goes beyond theoretical concepts and delves into practical applications.
Efficiency metrics play a pivotal role in evaluating the effectiveness of economic processes, policies, and models. In the context of econometrics, efficiency refers to the ability of a model to utilize resources optimally and produce accurate and reliable results. This is particularly crucial as econometric models are often used to analyze complex economic phenomena and make predictions based on historical data.
One of the fundamental efficiency metrics in econometrics is the accuracy of the model in predicting outcomes. Students are encouraged to utilize various statistical measures such as Mean Absolute Error (MAE), Mean Squared Error (MSE), and Root Mean Squared Error (RMSE) to assess the predictive performance of their models. These metrics provide insights into the degree of deviation between predicted values and actual observations, enabling students to refine their models for enhanced accuracy.
Furthermore, I guide students in adopting econometric methodologies that embrace efficiency as a multi-faceted concept. The stochastic frontier analysis (SFA) and data envelopment analysis (DEA) are two widely employed methodologies that measure efficiency in economic processes. SFA focuses on separating observed inefficiency from random error, providing a nuanced understanding of the factors influencing a model's performance. On the other hand, DEA assesses the relative efficiency of decision-making units, allowing students to compare different models or policies based on their resource utilization and output production.
To foster a holistic understanding of efficiency, students are encouraged to explore the intricacies of production, cost, and technical efficiency. Production efficiency involves maximizing output for a given level of input, while cost efficiency entails minimizing costs for a given level of output. Technical efficiency combines both aspects, providing a comprehensive view of how well resources are utilized in the production process. This approach equips students with the analytical tools to evaluate economic systems from various perspectives, contributing to a more nuanced interpretation of efficiency in econometrics.
In econometrics assignments, students often grapple with real-world data, introducing complexities that theoretical frameworks alone cannot address. As an expert guide, I stress the importance of robustness checks and sensitivity analyses to ensure the reliability of results in the face of data variability. By experimenting with different model specifications, variable inclusions, and econometric techniques, students can enhance the robustness of their findings and build confidence in the validity of their models.
Additionally, I encourage students to explore the concept of dynamic efficiency, recognizing that economic processes evolve over time. Time-series econometrics allows students to capture temporal patterns, trends, and shocks, providing a more accurate representation of the dynamic nature of economic phenomena. This approach equips students with the tools to analyze and predict changes in efficiency over time, offering valuable insights for policymakers and businesses adapting to evolving economic landscapes.
An expert's perspective on efficiency metrics and methodologies in econometrics extends beyond theoretical understanding to practical application. By emphasizing the significance of accuracy, comprehensive methodologies, and dynamic efficiency, students are equipped to navigate the complexities of econometrics assignments with a holistic and nuanced approach. This not only fosters a deeper understanding of the subject but also prepares students to contribute meaningfully to the field of economic analysis.
Analyzing Market Efficiency through Econometric Models
In the realm of econometrics, dissecting market efficiency through sophisticated models stands as a paramount pursuit. As an expert guiding students in unraveling the intricacies of econometric assignments, the crux lies in applying rigorous statistical methods to comprehend market dynamics. Econometric models serve as the compass, navigating through the labyrinth of data to discern patterns, relationships, and inefficiencies within markets.
Efficient Market Hypothesis (EMH) often takes center stage, postulating that asset prices embody all available information, rendering market participants unable to consistently achieve above-average returns. My guidance to students revolves around employing econometric tools such as regression analysis, time-series models, and event studies to scrutinize market anomalies and deviations from the EMH. Through meticulous examination of historical data, students uncover patterns that transcend the efficient market facade.
Furthermore, Monte Carlo simulations and GARCH models become invaluable tools in gauging market volatility, a critical aspect in comprehending efficiency. My expertise aids students in navigating the intricacies of these models, fostering a nuanced understanding of how economic variables interplay in the market ecosystem.
In essence, my role extends beyond teaching econometric techniques; it involves empowering students to unravel the market's complexity, equipping them with the analytical acumen to discern patterns, forecast trends, and contribute meaningfully to the discourse on market efficiency.
Risk Assessment in Financial Markets: A Quantitative Econometric Perspective
In the dynamic landscape of financial markets, understanding and managing risk is paramount for investors, traders, and financial institutions. The field of econometrics plays a crucial role in providing a quantitative perspective to assess and analyze risks in financial markets. As an expert guiding students through their econometrics assignments, it is imperative to delve into the intricacies of risk assessment, emphasizing the quantitative tools and methodologies that contribute to a comprehensive understanding of financial risk.
Econometrics, the intersection of economics, statistics, and mathematics, offers a robust framework for modeling and analyzing economic and financial data. When applied to financial markets, econometric techniques help in uncovering patterns, relationships, and trends that facilitate a quantitative assessment of risk.
One fundamental aspect of risk assessment in financial markets is the estimation of asset returns and volatility. Econometric models, such as the ARCH (Autoregressive Conditional Heteroskedasticity) and GARCH (Generalized Autoregressive Conditional Heteroskedasticity) models, are instrumental in capturing the time-varying nature of volatility. These models allow students to quantify and forecast market volatility, a crucial parameter in assessing risk.
Moreover, the Capital Asset Pricing Model (CAPM) and its extensions, often studied in econometrics assignments, provide insights into the systematic risk associated with an investment. By incorporating market risk measures, such as beta, students can assess how an asset's returns respond to overall market movements, aiding in the identification and quantification of systemic risk.
Value at Risk (VaR) is another indispensable tool in the econometric arsenal for risk assessment. Students exploring this concept learn to quantify the maximum potential loss within a specified confidence interval. By utilizing time series data and statistical distributions, econometric techniques enable the calculation of VaR, offering a quantitative measure of downside risk.
Beyond traditional models, students are introduced to copula functions and extreme value theory in econometrics assignments. These advanced techniques enhance risk assessment by capturing dependencies between financial variables and modeling tail events, which are crucial for understanding extreme market movements.
Challenges in Quantitative Risk Assessment:
While econometrics provides powerful tools for risk assessment, it is essential to acknowledge the challenges inherent in modeling financial markets. Non-stationarity, fat tails, and market microstructure issues pose hurdles that students must grapple with when applying quantitative econometric techniques.
Additionally, the ever-evolving nature of financial markets demands continuous adaptation of econometric models. As an expert guide, encouraging students to stay abreast of the latest developments in econometrics and financial market research ensures their ability to address emerging challenges and refine their risk assessment skills.
In the realm of financial markets, risk assessment is a complex yet vital endeavor. Through a quantitative econometric perspective, students gain a comprehensive understanding of the tools and methodologies employed in evaluating and managing risk. Whether estimating volatility, assessing systemic risk, or calculating Value at Risk, econometrics equips students with the skills necessary to navigate the intricate landscape of financial markets. As an expert providing guidance in econometrics assignments, fostering a deep appreciation for both the power and limitations of quantitative techniques ensures students develop the expertise required to make informed decisions in the dynamic world of finance.
Quantifying Uncertainty: Econometric Tools for Financial Risk Analysis
In the dynamic realm of financial markets, understanding and managing uncertainty is paramount. As an expert guiding students through Econometrics assignments, the focus lies on the intricate landscape of quantifying uncertainty through advanced tools for financial risk analysis. Econometric techniques serve as the bedrock for unraveling the complexities inherent in market fluctuations, enabling students to decipher and predict economic trends with precision.
The arsenal of tools includes robust statistical models, time series analysis, and volatility forecasting. These tools not only elucidate the past but also provide a lens into the future, aiding in risk assessment and strategic decision-making. Teaching students to wield econometric methods in financial risk analysis involves imparting the skills to model relationships between economic variables, estimate parameters, and critically evaluate the results.
GARCH models, and VaR (Value at Risk) computations become indispensable components of the student's toolkit. The expert guidance emphasizes not only technical proficiency but also the ability to interpret results in the context of real-world financial scenarios. It empowers students to navigate the turbulent waters of uncertainty, fostering a deeper understanding of the intricate interplay between economic factors. Ultimately, the expert mentor ensures that students emerge well-equipped to contribute meaningfully to the ever-evolving field of financial risk analysis.
Temporal Trends and Patterns: Harnessing Time Series Models for Financial Markets
In the dynamic realm of financial markets, understanding and predicting temporal trends and patterns is crucial for making informed investment decisions. Econometrics, the application of statistical methods to economic data, plays a pivotal role in unraveling the complexities inherent in financial time series data. As an expert guiding students through their Econometrics assignments, it becomes paramount to emphasize the significance of harnessing time series models to comprehend and forecast the intricacies of financial markets.
Financial markets are characterized by inherent volatility, influenced by a myriad of factors such as economic indicators, geopolitical events, and investor sentiment. Analyzing the temporal dimension of market data is essential to discern meaningful patterns and trends that can guide investment strategies. Time series models, a subset of econometric techniques, provide a powerful framework for studying the behavior of financial variables over time.
One of the fundamental challenges in financial econometrics is dealing with the inherent non-stationarity of financial time series data. Markets evolve, and their statistical properties change over time. As an expert, guiding students through their assignments involves imparting the knowledge of techniques like differencing or applying transformation methods to make the data stationary. Stationary time series are easier to model and can lead to more reliable forecasts, providing a solid foundation for understanding market dynamics.
A cornerstone of time series analysis is the autoregressive integrated moving average (ARIMA) model, a versatile tool for capturing temporal dependencies in financial data. ARIMA models involve differencing the data to achieve stationarity, followed by autoregressive (AR) and moving average (MA) components to model the temporal patterns. Teaching students to identify the appropriate order of differencing and the optimal ARIMA parameters equips them with the skills to model and forecast financial time series data effectively.
Beyond the conventional ARIMA models, the integration of machine learning techniques, such as neural networks and support vector machines, adds another layer of sophistication to the analysis. As an expert guiding students, it's essential to introduce the concept of deep learning models, emphasizing their ability to capture complex patterns and nonlinear relationships in financial data. However, it is crucial to highlight the importance of caution and thorough validation when deploying advanced machine learning models in a financial context, given the sensitivity of investment decisions.
Another critical aspect of financial time series analysis is the consideration of market efficiency and the presence of anomalies. Efficient Market Hypothesis (EMH) suggests that asset prices fully reflect all available information, making it challenging to consistently outperform the market. However, anomalies and inefficiencies do exist, and time series models help identify and exploit these opportunities. Teaching students to navigate the delicate balance between market efficiency and exploitable patterns is an integral part of guiding them through their Econometrics assignments.
The application of time series models in financial econometrics is indispensable for comprehending the temporal trends and patterns that drive market dynamics. As an expert assisting students, the emphasis should be on imparting a holistic understanding of both traditional and modern time series techniques. Equipping students with the skills to analyze, model, and interpret financial time series data empowers them to make informed decisions in the dynamic landscape of financial markets, thereby preparing them for success in their academic pursuits and future careers in finance.
Conclusion:
In conclusion, delving into the econometrics of financial markets offers a profound exploration into the intricate interplay between economic variables, market efficiency, and risk. As an expert guiding students in their econometrics assignments, it becomes evident that the realm of financial markets is not merely a confluence of numbers and statistical models but a dynamic ecosystem influenced by various factors.
The analysis of market efficiency, a cornerstone in econometrics, unveils the efficiency with which information is incorporated into asset prices. Efficient market hypothesis (EMH) forms the backbone of this exploration, asserting that prices fully reflect all available information. Through econometric tools and techniques, students gain a nuanced understanding of how market efficiency evolves over time and across different assets, providing them with a practical lens to assess investment strategies and decision-making processes.
Simultaneously, the examination of risk in financial markets requires a sophisticated econometric approach, wherein students grapple with volatility modeling, Value at Risk (VaR) estimation, and the application of advanced time-series methods. These aspects empower students to not only quantify risk but also comprehend the inherent uncertainties that pervade financial markets.
Furthermore, the econometrics of financial markets compels students to navigate the challenges posed by non-stationarity, endogeneity, and heteroscedasticity, among other econometric issues. The practical application of models like ARCH/GARCH, cointegration, and panel data techniques equips students with the analytical toolkit necessary to address these complexities and derive meaningful insights from financial data.
In guiding students through their econometrics assignments in this domain, the overarching goal is to foster a comprehensive understanding of the relationships between economic variables and market dynamics. Beyond the theoretical constructs, the emphasis lies in instilling practical skills that enable students to navigate real-world financial challenges with confidence.
In conclusion, the econometrics of financial markets transcends traditional academic boundaries, offering a holistic and dynamic perspective that mirrors the complexities of the financial world. As an expert assisting students in their econometrics journey, the aim is to cultivate not only technical proficiency but also a deep appreciation for the ever-evolving landscape of financial markets and the invaluable role econometrics plays in unraveling their intricacies.