Survival analysis in econometrics assignments is a pivotal aspect demanding precision and expertise. As an expert guiding students through these intricate studies, I emphasize techniques for duration and event studies, crucial for unraveling economic phenomena.
Duration analysis navigates the temporal dimension, evaluating the time until an event occurs. Whether analyzing the survival of firms in a competitive market or the persistence of unemployment, mastering duration models like Cox Proportional Hazard is imperative. These models illuminate covariate effects on survival probabilities, aiding students in understanding the intricate dynamics of economic variables over time.
Event studies, on the other hand, concentrate on the impact of specific occurrences on economic variables. From policy changes to market shocks, identifying causal relationships is intricate. Guiding students through methodologies like difference-in-differences or synthetic control methods fosters a nuanced comprehension of causal inference in econometrics.
Additionally, survival analysis requires a grasp of advanced statistical software. My guidance ensures students acquire proficiency in tools like R or Python, enabling them to implement these models effectively.
Survival analysis in econometrics assignments demands a comprehensive understanding of duration and event studies, statistical techniques, and programming proficiency. For those seeking assistance with their Econometrics assignment, my role as an expert is to illuminate these pathways, empowering students to dissect economic phenomena with analytical acumen and methodological finesse.
Foundations of Survival Analysis in Econometrics: Mastering Key Concepts for Duration and Event Studies
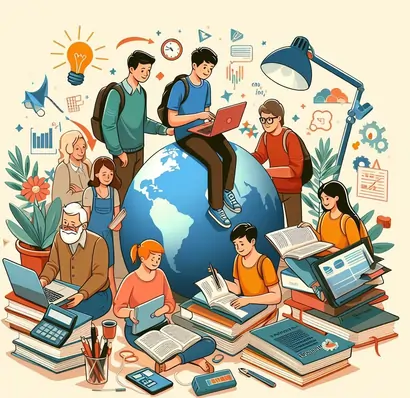
Survival analysis in econometrics is a powerful tool that allows researchers to examine the time until an event of interest occurs, providing invaluable insights into duration and event studies. As an expert guiding students through their econometrics assignments, it is crucial to impart a solid understanding of the foundations of survival analysis, enabling them to master key concepts and apply them effectively in their research endeavors.
At its core, survival analysis is particularly useful in economics for studying durations such as the time until unemployment, bankruptcy, or the failure of a firm. Events, in this context, represent the occurrence of an outcome that may influence the duration of interest. For example, in studying unemployment, the event could be finding a new job. Mastering survival analysis involves a comprehensive understanding of essential concepts, methodologies, and the underlying assumptions.
One fundamental concept is the hazard function, denoted by λ(t), which represents the instantaneous failure rate at time t given survival until that time. It provides a dynamic perspective on the likelihood of an event occurring at a specific point in time, capturing the time-varying nature of risks. Students must grasp the interpretation of the hazard function to gain insights into the changing dynamics of the studied phenomenon.
The survival function, denoted by S(t), complements the hazard function by representing the probability of surviving beyond time t. Students should recognize that S(t) and λ(t) are interconnected: as the survival probability decreases, the hazard rate increases. This relationship is fundamental in understanding the intricate dynamics of survival analysis.
Key statistical methodologies in survival analysis include Kaplan-Meier estimation and Cox proportional hazards regression. The Kaplan-Meier estimator is employed for non-parametric estimation of the survival function in the presence of censored data, where the exact time to the event is not observed for all subjects. The Cox proportional hazards model, on the other hand, is a powerful tool for analyzing the impact of covariates on the hazard function, allowing for the examination of multiple factors simultaneously.
Assumptions play a crucial role in survival analysis, and students need to be well-versed in these to ensure the validity of their results. Proportional hazards assumption is fundamental to the Cox model, implying that the hazard ratio between any two groups is constant over time. Violations of this assumption can lead to biased results, emphasizing the importance of careful model specification.
Furthermore, students should understand the implications of right-censoring, left-truncation, and other issues that may arise in real-world datasets. Addressing these challenges requires a nuanced approach and a solid foundation in both theoretical and applied aspects of survival analysis.
As an expert guiding students through their econometrics assignments, fostering a practical understanding of these concepts is paramount. Real-world applications and examples can enhance comprehension, illustrating how survival analysis contributes to addressing complex economic questions. Encouraging students to apply these techniques to their own research questions ensures a hands-on, experiential learning process.
Mastering the foundations of survival analysis in econometrics is essential for students aiming to conduct meaningful duration and event studies. As an expert mentor, providing clarity on concepts such as the hazard function, survival function, and statistical methodologies like Kaplan-Meier estimation and Cox proportional hazards regression is pivotal. Equipping students with the skills to navigate assumptions and challenges in real-world datasets ensures their ability to employ survival analysis effectively in their research pursuits. Ultimately, fostering a deep understanding of these key concepts empowers students to contribute meaningfully to the field of econometrics and make informed decisions in their analyses.
Survival Analysis Demystified: Essential Concepts for Econometrics Mastery
Survival Analysis, often perceived as a complex statistical technique, is demystified through a lens of expertise, illuminating essential concepts crucial for mastering econometrics assignments. As a guide for students navigating this intricate terrain, understanding the essence of Survival Analysis becomes paramount. Survival Analysis delves into time-to-event data, a cornerstone in econometrics, where events can be anything from economic transitions to market dynamics.
The expert discerns the fundamental concepts, emphasizing the Hazard Function as the linchpin. This function encapsulates the instantaneous failure rate at any given time, providing a nuanced perspective on the dynamics of economic events. Students are elucidated on censoring, a unique feature where some observations remain incomplete, mirroring real-world scenarios.
Kaplan-Meier estimators and Cox Proportional Hazards models emerge as indispensable tools in the econometric arsenal, aiding in analyzing survival patterns and covariate influences. The expert imparts a practical approach, bridging the gap between theoretical concepts and real-world applications, empowering students to unravel the intricacies of survival analysis in econometrics assignments.
In this demystification, the expert cultivates a transformative learning experience, equipping students with the acumen to navigate and conquer the challenges posed by survival analysis in the realm of econometrics.
Navigating Advanced Techniques in Econometric Survival Analysis: A Comprehensive Guide for Students
Econometric survival analysis, a powerful tool in economics, delves into the intricate dynamics of time-to-event data. As an expert in econometrics, I find immense satisfaction in guiding students through the maze of advanced techniques within survival analysis, equipping them with the skills needed to unravel complex economic phenomena. This comprehensive guide aims to illuminate the key concepts and methodologies, providing students with a roadmap to navigate the challenges inherent in econometric survival analysis.
- Introduction to Survival Analysis: Survival analysis extends beyond the realm of traditional econometrics, focusing on time until an event of interest occurs. This could range from the failure of a product to the time until an individual defaults on a loan. The complexity lies in handling censored data, where not all observations experience the event during the study period. Cox proportional hazards models and parametric survival models stand as the pillars of survival analysis, and a solid understanding of these is crucial for any student venturing into this domain.
- Cox Proportional Hazards Model: The Cox model is a cornerstone of survival analysis, offering a flexible and widely applicable framework. It allows for the exploration of covariate effects on the hazard function, enabling researchers to dissect the impact of various factors on the event of interest. My guidance emphasizes the nuanced interpretation of hazard ratios and the proper handling of time-varying covariates, ensuring that students grasp the intricacies of this fundamental model.
- Parametric Survival Models: Moving beyond the Cox model, parametric survival models provide a more explicit representation of the hazard function. We delve into models such as the Weibull, exponential, and log-logistic distributions, exploring their assumptions and implications. Through hands-on exercises, students gain proficiency in estimating parameters, conducting hypothesis tests, and validating model assumptions, preparing them to navigate real-world applications with confidence.
- Time-Dependent Covariates and Frailty Models: Survival analysis often involves covariates that change over time, adding a layer of complexity to the modeling process. My guidance emphasizes the proper handling of time-dependent covariates and introduces students to the concept of frailty models, which account for unobserved heterogeneity. This nuanced understanding is essential for capturing the subtleties of real-world data and refining the accuracy of survival predictions.
- Model Validation and Diagnostic Tools: Ensuring the reliability of survival models requires a keen eye for diagnostics. I guide students in employing diagnostic tools such as Kaplan-Meier curves, log-rank tests, and Schoenfeld residuals to assess model assumptions and identify potential pitfalls. Rigorous model validation is emphasized, promoting a holistic approach to econometric survival analysis that goes beyond mere parameter estimation.
- Practical Applications and Case Studies: To reinforce theoretical concepts, students are exposed to real-world applications and case studies. Analyzing survival data from diverse fields such as healthcare, finance, and engineering allows students to apply their skills in context, fostering a deeper understanding of the versatility and significance of econometric survival analysis.
Navigating advanced techniques in econometric survival analysis requires a blend of theoretical comprehension and practical application. Through this comprehensive guide, students are empowered to unravel the complexities of time-to-event data, equipping them with the skills needed to tackle real-world challenges. As an expert in econometrics, my commitment lies in fostering a generation of researchers capable of harnessing the full potential of survival analysis to contribute meaningfully to the field of economics.
Practical Applications of Survival Analysis: Enhancing Econometrics Assignments with Real-World Insights
Survival analysis, a statistical method originally developed in the field of medical research to analyze the time until an event of interest occurs, has found practical applications beyond its traditional domain. In recent years, econometrics, the branch of economics that applies statistical methods to test hypotheses and forecast future trends, has embraced survival analysis as a powerful tool for gaining insights into economic phenomena. As an expert guiding students through their econometrics assignments, integrating survival analysis into these tasks opens up a new dimension of real-world applications, enriching both the learning experience and the quality of analysis.
Survival analysis is particularly relevant in econometrics assignments when studying economic events that unfold over time, such as bankruptcy, unemployment, or the longevity of businesses. Incorporating survival analysis into these assignments enhances the depth and precision of the analysis by accounting for the dynamic nature of economic processes. For instance, when evaluating the sustainability of businesses, survival analysis allows students to model the time until a firm faces financial distress or closure, providing a more nuanced understanding of economic risks.
One practical application of survival analysis in econometrics assignments is the examination of labor market dynamics. Students can analyze the duration of unemployment spells or time until job placement for individuals, shedding light on the efficiency and resilience of labor markets. By incorporating real-world data into their assignments, students gain a more practical understanding of how economic theories manifest in actual employment scenarios, equipping them with valuable skills for future economic research or policy analysis.
Survival analysis also proves invaluable when exploring economic phenomena with temporal dependencies, such as financial crises. For example, students can use survival models to assess the duration until a country recovers from a recession or experiences a financial downturn. This not only enhances the predictive power of econometric models but also encourages students to critically assess the temporal aspects of economic events, recognizing that the time dimension is crucial in understanding economic dynamics.
In addition to its application in traditional econometrics assignments, survival analysis fosters a more interdisciplinary approach to economic research. Collaborating with experts from other fields, such as epidemiology or engineering, allows students to explore unconventional data sources and methodologies, enriching their analytical toolkit. This interdisciplinary perspective encourages a holistic understanding of economic phenomena, preparing students for the complex and interconnected challenges they may encounter in their future careers.
Moreover, survival analysis facilitates a more nuanced consideration of censored data, a common challenge in econometrics when dealing with incomplete information. Students learn to handle situations where the exact timing of an economic event is unknown or unavailable, a skill that proves invaluable when working with real-world data. This enhances their ability to navigate uncertainties and make informed decisions in the face of incomplete information, a vital skill in both academic and professional settings.
The integration of survival analysis into econometrics assignments brings a wealth of practical applications and real-world insights. As an expert guiding students through these assignments, encouraging the adoption of survival analysis enhances their analytical skills, prepares them for interdisciplinary collaboration, and equips them with tools to handle complex, real-world economic challenges. By bridging the gap between theory and application, survival analysis enriches the econometrics learning experience, empowering students to become more adept and insightful economists in their future careers.
Conclusion:
In conclusion, the exploration of survival analysis in econometrics assignments, focusing on techniques for duration and event studies, reveals a critical dimension in understanding economic phenomena with a temporal perspective. As an expert guiding students through their business economics assignments, it is evident that survival analysis offers a robust framework for modeling and analyzing the time until an event of interest occurs.
The utilization of survival analysis techniques allows students to delve into the intricate dynamics of economic variables, capturing the duration until an event takes place or an economic state changes. The significance of incorporating such methods in econometrics assignments lies in their ability to provide a nuanced understanding of time-dependent processes, offering insights that may not be apparent through traditional analyses.
Moreover, survival analysis equips students with a versatile set of tools, ranging from Kaplan-Meier estimators to Cox proportional hazards models, enabling them to address diverse research questions. This adaptability is crucial in the realm of business economics, where the timing and occurrence of events often play a pivotal role in shaping economic outcomes.
Furthermore, the application of survival analysis fosters a deeper appreciation for the real-world applicability of econometric techniques. By incorporating time-to-event considerations, students gain a more comprehensive understanding of economic behaviors and can better navigate the complexities of dynamic systems.
In guiding students through assignments in business economics, emphasizing the importance of survival analysis encourages a more sophisticated approach to modeling economic phenomena. It challenges students to think beyond static frameworks and embrace the temporal dimension inherent in economic processes.
In conclusion, survival analysis techniques stand as valuable tools for students venturing into econometrics assignments in business economics. As an expert, the encouragement of exploring these methods empowers students to unravel the intricacies of time-dependent economic dynamics, ultimately enhancing their analytical skills and contributing to a more holistic comprehension of economic systems.