- A Comprehensive Exploration of Machine Learning Techniques for Unprecedented Analytical Precision in Economic Data
- The Evolution of Econometrics and Machine Learning
- Supervised Learning for Economic Predictions
- Unleashing the Potential of Unsupervised Learning
- Time Series Analysis and Forecasting
- Overcoming Challenges with Ensemble Methods
- Ethical Considerations and Model Interpretability
- Tracing the Integration Path from Econometrics to Machine Learning Paradigms
- Machine Learning Applications in Complex Economic Modeling and Forecasting
- Foundations of Machine Learning in Economics:
- Handling Big Data:
- Non-Linearity and Complex Relationships:
- Forecasting Accuracy and Precision:
- Risk Management and Decision Support:
- Challenges and Considerations:
- Unraveling the Intricacies of Economic Dynamics with Cutting-Edge Machine Learning Models
- Addressing Complex Challenges in Implementing Machine Learning Techniques for Econometric Analyses
- A Thoughtful Examination of the Ethical Implications Surrounding Machine Learning in Econometrics
- Conclusion:
In the dynamic realm of econometrics, the integration of machine learning (ML) has ushered in a new era of sophisticated data analysis, reshaping the landscape for students navigating the complexities of economic modeling. As an expert guiding students through their econometrics assignments, it is imperative to illuminate the transformative impact of modern ML approaches. Whether you require help with your econometrics assignment or seek to understand the evolving methodologies in economic modeling, exploring the integration of machine learning techniques enhances students' capabilities in analyzing economic data and making informed decisions.
Machine learning empowers econometricians to grapple with vast datasets and intricate relationships that traditional methods may struggle to capture. Algorithms such as neural networks, support vector machines, and ensemble methods provide a robust toolkit for modeling non-linearities, intricate dependencies, and latent patterns within economic data. This departure from conventional econometric techniques widens the analytical scope, allowing students to discern nuanced economic phenomena and enhance the accuracy of predictions.
The expert mentor must facilitate a nuanced understanding of ML techniques, elucidating their applications in econometrics. Emphasizing the importance of feature engineering, model selection, and interpretation of results becomes paramount. Moreover, guiding students on the ethical considerations and potential biases in ML models is crucial, instilling a holistic approach to economic data analysis.
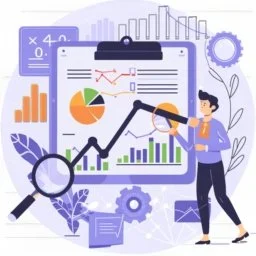
The synergy between machine learning and econometrics marks a paradigm shift, offering students a powerful arsenal to dissect and comprehend economic intricacies. Nurturing a comprehensive understanding of these modern approaches ensures that aspiring econometricians not only complete assignments proficiently but also contribute to the advancement of economic research and policy.
A Comprehensive Exploration of Machine Learning Techniques for Unprecedented Analytical Precision in Economic Data
In the dynamic landscape of economic analysis, the integration of machine learning techniques has become imperative to unlock unprecedented analytical precision. As an expert guiding students through their econometrics assignments, it is essential to delve into the realm of machine learning and its transformative impact on the interpretation and forecasting of economic data.
The Evolution of Econometrics and Machine Learning
Econometrics, traditionally rooted in statistical methods, has witnessed a paradigm shift with the emergence of machine learning. While traditional econometric models rely on explicit assumptions and linear relationships, machine learning algorithms excel in capturing complex, nonlinear patterns inherent in economic data. As educators, we must equip students with the knowledge to navigate this intersection between econometrics and machine learning for more robust analyses.
Supervised Learning for Economic Predictions
Supervised learning techniques, such as linear regression, decision trees, and support vector machines, offer students powerful tools for predicting economic outcomes. By training models on historical data, these algorithms learn patterns and relationships, enabling more accurate predictions of economic indicators. It is crucial for students to understand the nuances of model selection, feature engineering, and hyperparameter tuning to harness the full potential of supervised learning in economic analysis.
Unleashing the Potential of Unsupervised Learning
Unsupervised learning, exemplified by clustering and dimensionality reduction techniques, contributes significantly to uncovering hidden structures within economic datasets. Students can employ methods like k-means clustering to identify distinct economic segments or principal component analysis (PCA) to reduce the dimensionality of variables, revealing underlying patterns that traditional econometric approaches may overlook.
Time Series Analysis and Forecasting
Economic data often exhibits temporal dependencies, making time series analysis a critical component of econometrics assignments. Machine learning techniques, including autoregressive integrated moving average (ARIMA) models and recurrent neural networks (RNNs), provide advanced tools for forecasting economic trends. Educators should guide students in understanding the intricacies of time series data, model selection, and the importance of considering lagged variables for accurate predictions.
Overcoming Challenges with Ensemble Methods
Ensemble methods, such as random forests and gradient boosting, empower students to address challenges like overfitting and enhance the robustness of their models. By combining the strength of multiple algorithms, ensemble methods offer a more comprehensive and accurate representation of economic relationships. Educators should emphasize the importance of model diversity and the delicate balance between bias and variance in achieving optimal predictive performance.
Ethical Considerations and Model Interpretability
As students explore machine learning techniques, it is crucial to instill an awareness of ethical considerations. Understanding the potential biases embedded in data and models is essential for responsible economic analysis. Additionally, the interpretability of machine learning models remains a critical aspect. Educators should guide students in adopting techniques like LIME (Local Interpretable Model-agnostic Explanations) to enhance model transparency and facilitate a deeper understanding of the economic implications of their analyses.
In the ever-evolving landscape of economic analysis, the incorporation of machine learning techniques elevates the precision and depth of insights derived from data. As educators guiding students through their econometrics assignments, it is our responsibility to impart the knowledge and skills necessary to navigate this intersection seamlessly. By embracing the synergy between traditional econometrics and cutting-edge machine learning, students can unlock unparalleled analytical precision and contribute meaningfully to the advancement of economic understanding.
Tracing the Integration Path from Econometrics to Machine Learning Paradigms
Navigating the evolution from traditional econometrics to contemporary machine learning paradigms is akin to embarking on a transformative journey in quantitative analysis. As an expert guiding students through their econometrics assignments, I witness the profound shift in methodologies. The integration path is a dynamic trajectory that witnesses the fusion of statistical rigor with the computational prowess of machine learning.
Econometrics, rooted in statistical methods, laid the foundation for understanding economic phenomena. However, as complexities grew, the limitations of traditional models became apparent. The infusion of machine learning into econometric frameworks marks a pivotal paradigm shift. Students must now grasp not only the intricacies of econometric models but also the algorithms underpinning machine learning.
This integration path unfolds with students acquiring a dual skill set – a nuanced comprehension of econometric principles and a mastery of machine learning techniques. The econometrician of today must navigate through regression analysis, time series modeling, and causal inference, while also harnessing the predictive power of machine learning algorithms like neural networks and ensemble methods.
Guiding students through this integration demands an emphasis on interdisciplinary knowledge. The expert facilitator must illuminate the synergies between econometrics and machine learning, fostering an appreciation for the strengths each approach brings. Ultimately, this journey empowers students to wield a comprehensive toolkit, enhancing their analytical capabilities and preparing them for the evolving landscape of quantitative analysis in economics.
Machine Learning Applications in Complex Economic Modeling and Forecasting
In the dynamic landscape of economics, traditional econometric models face challenges in capturing the intricate relationships within complex economic systems. The advent of machine learning (ML) techniques has revolutionized the field, offering unprecedented capabilities in handling large datasets, non-linear relationships, and intricate patterns. As an expert guiding students through their econometrics assignments, understanding and integrating machine learning applications is crucial for comprehending and predicting complex economic phenomena.
Foundations of Machine Learning in Economics:
Machine learning encompasses a diverse set of algorithms designed to learn patterns and make predictions without explicit programming. In the realm of economics, where traditional models may fall short, ML techniques offer a powerful alternative. Regression models, decision trees, neural networks, and ensemble methods are just a few examples of ML tools that can be applied to economic data.
Handling Big Data:
One significant advantage of machine learning in economic modeling is its ability to handle vast amounts of data. Economic systems generate an immense volume of information, and ML algorithms excel at extracting meaningful insights from these datasets. Whether dealing with macroeconomic indicators, financial market data, or consumer behavior, ML provides a scalable solution to process and analyze information efficiently.
Non-Linearity and Complex Relationships:
Economic systems often exhibit non-linear relationships and intricate dependencies that traditional econometric models struggle to capture. Machine learning models, particularly neural networks, are well-suited for handling non-linearity. They can uncover hidden patterns, adapt to complex structures, and model relationships that may not be apparent through linear regression or other traditional techniques.
Forecasting Accuracy and Precision:
Accurate economic forecasting is at the core of econometrics. Machine learning algorithms enhance forecasting precision by leveraging historical data to identify patterns and trends. Time-series models, recurrent neural networks, and Long Short-Term Memory (LSTM) networks are examples of ML techniques that excel in capturing temporal dependencies, improving the accuracy of economic predictions.
Risk Management and Decision Support:
In the realm of economic decision-making, machine learning plays a crucial role in risk management. Models can assess the impact of various economic factors on specific outcomes, aiding policymakers, businesses, and investors in making informed decisions. Monte Carlo simulations, for instance, use ML algorithms to simulate numerous scenarios, providing insights into potential risks and uncertainties.
Challenges and Considerations:
While machine learning offers powerful tools for economic modeling, it is essential to acknowledge the challenges. Overfitting, data quality issues, and interpretability concerns are among the considerations that must be addressed. As an expert guiding students through econometrics assignments, emphasis should be placed on understanding the assumptions and limitations of different ML models to ensure robust and reliable results.
As the field of economics continues to evolve, incorporating machine learning applications into econometric analysis becomes imperative. Students working on econometrics assignments should be equipped with the knowledge and skills to leverage ML techniques effectively. By embracing the power of machine learning in handling big data, capturing non-linear relationships, and improving forecasting accuracy, students can enhance their ability to model and predict complex economic phenomena, contributing to the advancement of economic research and decision-making.
Unraveling the Intricacies of Economic Dynamics with Cutting-Edge Machine Learning Models
In the realm of economic dynamics, the integration of cutting-edge machine learning models has emerged as a transformative force, transcending traditional econometric approaches. As an expert guiding students through the labyrinth of econometrics assignments, I witness the evolving landscape where sophisticated algorithms unravel the intricacies of economic phenomena.
Machine learning, with its ability to analyze vast datasets and discern complex patterns, offers a nuanced understanding of economic dynamics. Students are now equipped with tools that surpass the limitations of classical econometric methods, allowing them to explore intricate relationships, forecast trends, and make informed policy recommendations. Through the lens of machine learning, econometrics assignments become an exploration of predictive analytics, clustering techniques, and deep learning applications tailored to economic contexts.
The synergy of economic theory and machine learning is evident as students delve into regression models enriched with neural networks, time series analysis empowered by recurrent neural networks, and the interpretation of economic shocks through advanced clustering algorithms. By embracing these technological advancements, students not only master econometric principles but also gain a competitive edge in addressing real-world economic challenges.
As an expert facilitator, witnessing the symbiosis of economic theory and cutting-edge technology in students' assignments is both gratifying and inspiring. Nurturing a generation adept at unraveling economic intricacies through innovative machine learning models ensures that the future of economic analysis is dynamic, adaptive, and truly insightful.
Addressing Complex Challenges in Implementing Machine Learning Techniques for Econometric Analyses
In the realm of econometrics, the integration of machine learning techniques has emerged as a transformative force, offering unparalleled insights and predictive capabilities. As an expert guiding students through the intricate landscape of econometric assignments, addressing the complex challenges associated with implementing machine learning in this domain becomes paramount.
Machine learning, with its ability to decipher patterns and extract meaningful information from vast datasets, promises to revolutionize econometric analyses. However, the convergence of these two disciplines is not without hurdles. From conceptual understanding to practical implementation, students often grapple with various intricacies when incorporating machine learning into their econometric assignments.
First and foremost, bridging the gap between traditional econometric methods and machine learning frameworks requires a solid foundation in both domains. Students must comprehend the theoretical underpinnings of econometric models while simultaneously mastering the diverse algorithms and methodologies inherent in machine learning. Establishing this dual expertise is crucial for seamlessly integrating the strengths of both approaches to yield more robust and accurate analyses.
Furthermore, students encounter challenges in selecting the appropriate machine learning model for a given econometric problem. The plethora of algorithms, ranging from linear regression to sophisticated neural networks, necessitates a nuanced understanding of the dataset and research question at hand. Educating students about the strengths, limitations, and assumptions associated with each model empowers them to make informed choices tailored to the unique characteristics of their econometric assignments.
Data quality and preprocessing present additional hurdles in the application of machine learning to econometric analyses. Real-world datasets often exhibit complexities, such as missing values, outliers, and non-linearity, which can significantly impact model performance. Students must acquire skills in data cleaning, feature engineering, and normalization to ensure the robustness and reliability of their machine learning-based econometric models.
In the dynamic field of econometrics, staying abreast of technological advancements is crucial. Continuous updates in machine learning algorithms and tools demand that students remain vigilant in updating their skill sets. As an expert guiding students, it becomes imperative to emphasize the importance of ongoing education and the incorporation of emerging technologies to enhance the efficacy of econometric analyses.
Collaboration between econometricians and machine learning experts becomes essential in addressing these challenges. Encouraging interdisciplinary teamwork facilitates the exchange of knowledge and expertise, ensuring that students are equipped with a holistic skill set that spans both econometrics and machine learning. This collaborative approach enables students to navigate the complexities of implementing machine learning techniques in econometric analyses effectively.
Moreover, a nuanced understanding of model evaluation and interpretation is pivotal. Students often grapple with the intricacies of assessing the performance of machine learning models in the econometric context. Concepts like overfitting, bias-variance tradeoff, and cross-validation require careful consideration to avoid misinterpretations and ensure the reliability of results.
The intricate process of implementing machine learning techniques for econometric analyses requires a comprehensive and interdisciplinary approach. Addressing the challenges associated with merging these two domains involves imparting dual expertise, navigating data complexities, staying abreast of technological advancements, fostering collaboration, and honing model evaluation skills. As an expert, nurturing these competencies equips students with the tools necessary to harness the full potential of machine learning in the realm of econometrics, ultimately paving the way for more accurate and insightful analyses.
A Thoughtful Examination of the Ethical Implications Surrounding Machine Learning in Econometrics
In the ever-evolving landscape of econometrics, the integration of machine learning (ML) techniques raises profound ethical considerations that demand thoughtful examination. As an expert guiding students through their econometrics assignments, I am acutely aware of the ethical implications surrounding the intersection of human decision-making and machine-driven analytics.
Machine learning algorithms, while powerful tools for predictive modeling, introduce complexities in ethical decision-making. One paramount concern is the potential for bias inherent in the data used to train these models. As students delve into econometric assignments, it is crucial to explore and address the ethical dimensions of selecting, preprocessing, and interpreting data, as biased inputs can perpetuate and exacerbate societal inequalities.
Transparency and interpretability in machine learning models are additional ethical challenges. Students must grapple with the trade-off between predictive accuracy and the ability to comprehend and explain model outputs. A thorough exploration of the ethical implications involves guiding students to consider the broader societal impact of opaque algorithms, particularly in economic decision-making where transparency is vital.
Moreover, the ethical responsibility extends to ensuring that machine learning applications in econometrics adhere to privacy norms and data protection regulations. Students must navigate the delicate balance between extracting meaningful insights and safeguarding individual privacy.
Ultimately, a comprehensive examination of the ethical implications surrounding machine learning in econometrics empowers students not only to master the technical aspects of their assignments but also to cultivate a nuanced understanding of the broader societal implications of their work. This approach fosters a generation of econometricians who are not only proficient in their craft but also ethically conscious stewards of data-driven decision-making.
Conclusion:
In conclusion, the intersection of machine learning and econometrics has ushered in a new era of sophisticated and nuanced economic data analysis. As an expert guiding students in completing their econometrics assignments, it is evident that the incorporation of modern machine learning approaches has significantly enhanced the toolkit available for extracting meaningful insights from economic data.
Machine learning techniques, ranging from traditional regression models to advanced algorithms like neural networks and ensemble methods, offer a versatile set of tools that can capture complex patterns and relationships within economic datasets. This not only facilitates more accurate predictions but also enables economists to uncover latent factors that might have been overlooked by traditional econometric methods.
Furthermore, the synergy between machine learning and econometrics has proven instrumental in addressing challenges such as non-linearity, multicollinearity, and high dimensionality that often characterize economic data. The ability of machine learning models to handle large datasets and complex interactions allows for a more realistic representation of the dynamic economic systems under study.
However, it is crucial to approach the integration of machine learning in econometrics with a judicious balance. While the power and flexibility of machine learning models offer tremendous advantages, the interpretability of results remains a concern. As an expert guide, emphasis should be placed on understanding the underlying assumptions of the chosen models and the implications of their outcomes in the context of economic theory.
In guiding students, it is important to instill a comprehensive understanding of both traditional econometric methods and modern machine learning techniques. This ensures that students not only leverage the cutting-edge tools available but also appreciate the theoretical foundations that underpin economic analysis.
In essence, the amalgamation of machine learning and econometrics heralds an exciting era in economic data analysis. As an expert mentor, fostering a balanced and informed approach allows students to harness the full potential of these modern approaches while upholding the core principles of econometrics. The future of economic research and policy analysis lies in the hands of those who can seamlessly navigate the synergies between these two domains, pushing the boundaries of knowledge in the ever-evolving field of economics.