- Panel Data Analysis: Unraveling Temporal Dynamics in Microeconometrics
- Exploring Longitudinal Patterns: A Comprehensive Guide to Panel Data Models
- Instrumental Variables and Causal Inference: Untangling Complex Relationships in Microeconometrics
- Navigating Endogeneity Challenges: Mastering Instrumental Variable Method
- Discrete Choice Models: Understanding Decision-Making at the Micro Level
- Deciphering Preferences: Applications and Interpretations of Discrete Choice Models
- Conclusion:
Microeconometric techniques play a pivotal role in understanding and analyzing individual and firm behavior within the realm of economics. As an expert guiding students through their Econometrics assignments, it is crucial to emphasize the significance of these techniques in unraveling intricate economic relationships at the micro level. If you need assistance with your econometrics assignment, don't hesitate to reach out. I'm here to provide support and guidance to ensure your success.
Students delve into a myriad of methods, ranging from basic regression analysis to advanced models like instrumental variable estimation and panel data analysis. Each technique serves a unique purpose, allowing students to discern the factors influencing individual and firm decisions. For instance, by employing discrete choice models, students can explore the determinants of consumer preferences or hiring choices made by firms.
The intricacies of microeconometric techniques empower students to grapple with real-world economic problems. Through hands-on assignments, they learn to navigate issues such as endogeneity, sample selection, and heteroscedasticity, gaining practical skills to dissect complex economic phenomena.
Moreover, understanding individual and firm behavior is crucial for policymakers and businesses. Students equipped with microeconometric expertise can contribute meaningfully to evidence-based decision-making. As an expert guide, my role involves not just imparting technical know-how but also instilling a problem-solving mindset, preparing students to tackle the nuanced challenges of analyzing microeconomic data with precision and insight.
Panel Data Analysis: Unraveling Temporal Dynamics in Microeconometrics
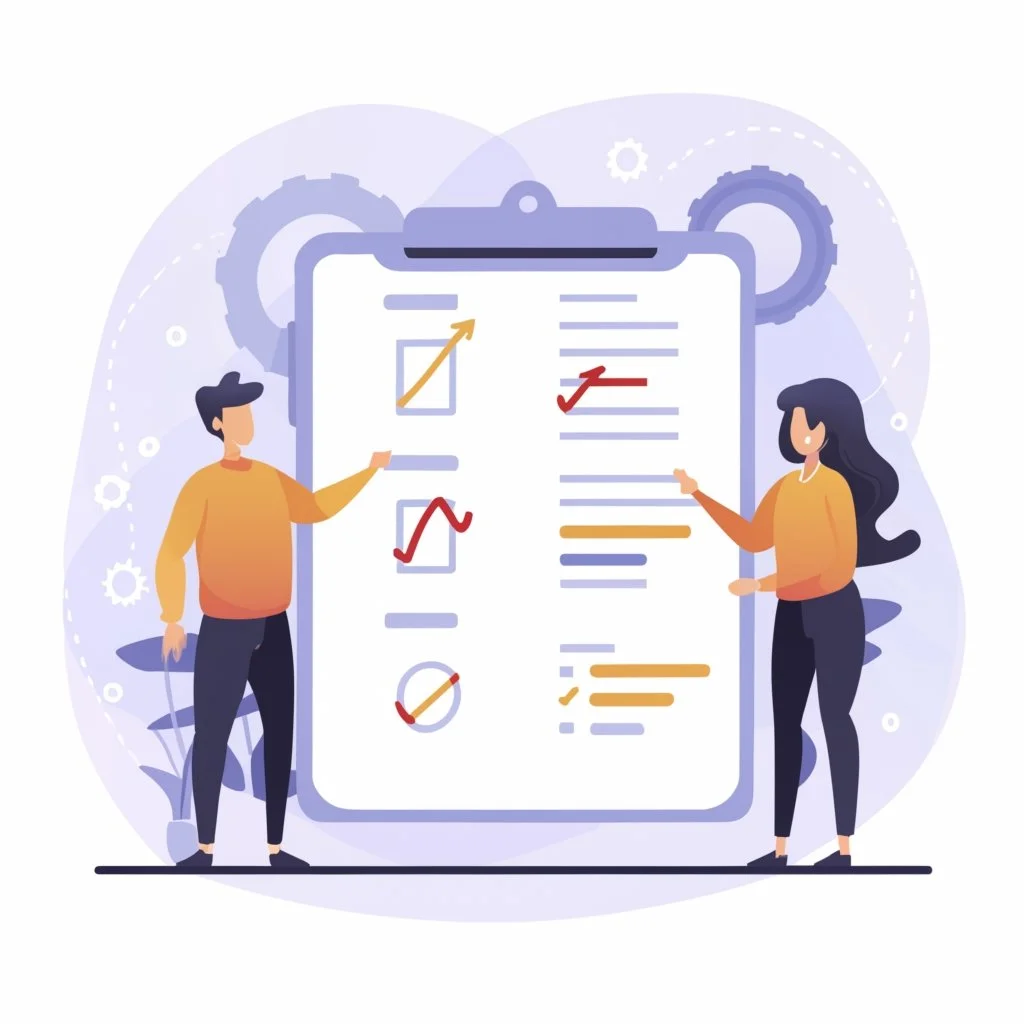
As an expert guiding students through the intricate realm of econometrics, I understand the pivotal role that panel data analysis plays in unraveling the temporal dynamics inherent in microeconometrics. This advanced statistical method has become indispensable for researchers and policymakers seeking a deeper understanding of how individual entities evolve over time, making it a cornerstone in the study of economic phenomena.
Panel data, also known as longitudinal or cross-sectional time-series data, involves observations on multiple entities over multiple time periods. It provides a unique opportunity to explore both individual and temporal variations, allowing for a more nuanced and comprehensive analysis compared to traditional cross-sectional or time-series data alone. In the world of microeconometrics, where the focus is on individual units such as firms, households, or individuals, panel data analysis becomes a powerful tool for capturing the complexity of economic behavior.
One of the primary advantages of panel data lies in its ability to control for individual heterogeneity. In microeconometrics, individuals or entities may exhibit differences that persist over time, influencing their economic behavior. These differences, often termed fixed effects, can be effectively accounted for in panel data models. By doing so, researchers can disentangle the impact of time-varying factors from the inherent characteristics of individual entities, providing more accurate and reliable estimates.
Temporal dynamics play a crucial role in understanding economic phenomena, as they capture the evolution of variables over time. This is particularly pertinent in microeconometrics, where the focus is on how individual units respond to changing economic conditions. Panel data analysis allows for the identification and estimation of dynamic relationships, shedding light on the lagged effects, persistence, and adjustment processes that characterize economic behavior. For students delving into econometrics assignments, mastering these techniques is essential for conducting robust empirical analyses.
The dynamic panel data models, such as the Arellano-Bond model, facilitate the examination of lagged effects and endogeneity concerns. These models acknowledge the interplay between current and past observations, offering a comprehensive framework for studying economic dynamics. As an expert mentor, I guide students through the intricacies of specifying and estimating dynamic panel data models, emphasizing the importance of addressing endogeneity and the potential biases introduced by unobserved heterogeneity.
Furthermore, panel data analysis allows for the exploration of individual responses to policy interventions or external shocks. By incorporating fixed effects and time-varying covariates, researchers can assess the impact of policy changes on different entities over time, providing valuable insights for policymakers. This aspect of panel data analysis is particularly relevant in microeconometrics, where understanding the heterogeneous effects of interventions on individuals or firms is crucial for informed decision-making.
In guiding students through their econometrics assignments, I emphasize the significance of selecting appropriate panel data models, addressing potential econometric issues, and interpreting results in the context of economic theory. It is crucial for students to grasp the nuances of panel data analysis, as it not only enhances their analytical skills but also equips them with the tools needed to contribute meaningfully to the field of microeconometrics.
Panel data analysis stands as a cornerstone in unraveling the temporal dynamics of microeconometrics. Its ability to capture individual heterogeneity, dynamic relationships, and responses to policy changes makes it an invaluable tool for researchers and policymakers alike. As an expert guiding students in their econometrics assignments, my focus is on imparting the knowledge and skills necessary to navigate the complexities of panel data analysis, empowering students to conduct robust and insightful analyses in the ever-evolving landscape of microeconometrics.
Exploring Longitudinal Patterns: A Comprehensive Guide to Panel Data Models
As an expert guiding students through the intricate realm of econometrics, delving into the exploration of longitudinal patterns is a crucial facet of understanding and applying panel data models. This comprehensive guide aims to unravel the intricacies of longitudinal data analysis, providing students with a robust foundation for their econometrics assignments.
Longitudinal patterns, captured through repeated observations over time on the same units, present a rich source of information for econometric analyses. Panel data models, adept at handling such longitudinal datasets, empower students to uncover dynamic relationships and temporal dependencies within economic phenomena. This guide emphasizes the significance of mastering fixed effects, random effects, and first-difference models, elucidating their distinctive applications in capturing individual and time-specific variations.
Navigating the nuances of panel data estimation techniques, students are equipped to make informed choices based on the nature of their data and research questions. The guide encourages the exploration of heterogeneity, endogeneity, and issues related to model specification, fostering a holistic understanding of the challenges inherent in longitudinal analyses.
By demystifying the intricacies of panel data models, this guide not only facilitates academic success in econometrics assignments but also cultivates a deeper appreciation for the temporal dynamics inherent in economic phenomena. As students grasp the intricacies of longitudinal patterns, they embark on a journey towards becoming adept econometricians, capable of unraveling the complexities of real-world economic data.
Instrumental Variables and Causal Inference: Untangling Complex Relationships in Microeconometrics
In the intricate realm of microeconometrics, navigating the labyrinth of complex relationships between variables often requires advanced statistical tools and methodologies. One such powerful tool that aids in unraveling causal relationships is instrumental variables (IV) analysis. As an expert guiding students through their econometrics assignments, I find myself emphasizing the significance of instrumental variables as a cornerstone for establishing causal inference in observational studies.
At its core, microeconometrics seeks to understand the impact of changes in one variable on another within a specific economic context. However, establishing causality in observational data is challenging due to the presence of confounding variables and potential endogeneity issues. This is where instrumental variables come into play, offering a methodological refuge for researchers aiming to disentangle complex relationships.
Instrumental variables serve as a bridge between econometric theory and real-world applications, providing a robust framework for addressing endogeneity concerns. Endogeneity arises when an explanatory variable is correlated with the error term, leading to biased and inconsistent parameter estimates. This phenomenon can jeopardize the validity of causal inferences drawn from statistical analyses. Instrumental variables act as an antidote by introducing an exogenous variable that is correlated with the endogenous variable of interest but is not directly related to the dependent variable, allowing researchers to isolate the causal effect.
In the context of guiding students through their econometrics assignments, I emphasize the careful selection of instrumental variables. A valid instrument must satisfy two essential criteria: relevance and exogeneity. Relevance ensures that the instrument is correlated with the endogenous variable, while exogeneity ensures that the instrument is not directly related to the dependent variable, except through its impact on the endogenous variable. This meticulous selection process is crucial for the success of instrumental variable analysis and the credibility of causal inferences.
The practical application of instrumental variables is demonstrated through various econometric models, such as the two-stage least squares (2SLS) estimation method. In the first stage, the instrument is used to predict the endogenous variable, and in the second stage, the predicted values are utilized in the main regression equation. This two-step process mitigates endogeneity concerns and provides consistent estimates of the causal relationship.
Furthermore, guiding students in econometrics assignments involves imparting a nuanced understanding of potential pitfalls and challenges associated with instrumental variable analysis. Students must be aware of over-identification tests to assess the validity of instruments and the importance of considering instrument relevance throughout the analysis. Clear communication of these concepts is essential to equip students with the skills necessary to navigate the intricacies of microeconometrics.
As an expert in the field, I stress the importance of robustness checks and sensitivity analyses in econometric assignments utilizing instrumental variables. Sensitivity to instrument validity and potential sources of bias ensures that students develop a comprehensive and critical approach to their analyses, fostering a deeper understanding of the limitations and assumptions inherent in causal inference through instrumental variables.
Instrumental variables stand as a crucial tool in the econometrician's arsenal, offering a methodological lifeline for disentangling complex relationships in microeconometrics. Guiding students through the intricacies of instrumental variable analysis not only enhances their analytical skills but also equips them with a deeper appreciation for the challenges and opportunities presented by causal inference in the dynamic world of economic research. As educators, it is our responsibility to cultivate a generation of econometricians who can confidently navigate the complexities of microeconometrics, armed with the powerful tool of instrumental variables.
Navigating Endogeneity Challenges: Mastering Instrumental Variable Method
As an experienced guide in the realm of econometrics, mastering the instrumental variable (IV) method is a crucial skill when navigating the intricate landscape of endogeneity challenges. Endogeneity, a pervasive issue in empirical research, arises when a variable of interest is correlated with the error term, leading to biased and inconsistent parameter estimates. In the pursuit of precision and accuracy, students undertaking econometrics assignments often encounter the need for robust methodologies to overcome endogeneity hurdles.
The instrumental variable method serves as a potent tool in this endeavor, offering a systematic approach to disentangle causation from correlation. Students are coached to meticulously select instrumental variables that meet the crucial criteria of relevance and exogeneity, ensuring the integrity of the instrument in isolating the endogenous regressor. Understanding the intricacies of instrument validation and the underlying assumptions becomes paramount in wielding this method effectively.
Expert guidance involves imparting not just theoretical knowledge but also practical insights into identifying suitable instruments within the context of specific economic models. Clear explanations on the importance of instrument strength and potential pitfalls in IV estimation enhance students' capacity to apply this method with confidence. Nurturing a deep understanding of IV method nuances empowers students to tackle endogeneity challenges head-on, fostering a foundation for sound econometric analysis and contributing to their academic and professional growth.
Discrete Choice Models: Understanding Decision-Making at the Micro Level
One area that frequently captures the attention of budding econometricians is discrete choice models. These models are pivotal in understanding decision-making at the micro level, offering a lens through which we can analyze and predict choices made by individuals or entities in various scenarios.
Discrete choice models are a subset of econometric models designed to study decisions involving distinct, mutually exclusive alternatives. Whether it's consumers choosing between different brands, individuals deciding on transportation modes, or firms selecting production technologies, discrete choice models provide a powerful framework to analyze such decisions.
At the heart of these models lies the concept of utility, a measure of satisfaction or preference that individuals derive from their choices. The fundamental assumption is that individuals aim to maximize their utility when making decisions. This optimization process is formalized through mathematical expressions, paving the way for a rigorous econometric analysis.
One of the fundamental models within this category is the multinomial logit model (MNL), a workhorse in discrete choice analysis. This model assumes that the probability of choosing a particular alternative is determined by the exponential of a linear combination of explanatory variables, capturing the influence of various factors on the decision-making process. The MNL model provides a robust foundation for understanding and estimating choices across different alternatives.
Beyond the MNL model, researchers and students delve into more advanced frameworks like the nested logit model, mixed logit model, and the panel data models for repeated observations on individuals or entities over time. These models allow for a richer exploration of decision-making, accommodating complexities such as correlated choices and heterogeneity among decision-makers.
To successfully tackle econometrics assignments related to discrete choice models, students need to grasp the underlying assumptions, mathematical formulations, and estimation techniques associated with these models. Econometric software such as Stata, R, or Python becomes an indispensable tool for implementing these models, as it facilitates parameter estimation, hypothesis testing, and model diagnostics.
One of the critical challenges in discrete choice modeling lies in handling endogeneity and unobserved heterogeneity. These issues can significantly impact the reliability of estimates, making it essential for students to employ rigorous econometric methods to address these challenges. Instrumental variable approaches, fixed-effects models, and random effects models are among the techniques employed to mitigate these issues.
The real-world applications of discrete choice models are diverse and impactful. For instance, policymakers can use these models to predict the likely adoption of new policies, marketing professionals can optimize product offerings based on consumer preferences, and transportation planners can design more efficient and user-friendly systems.
Discrete choice models are a cornerstone of microeconometric analysis, allowing economists to gain deep insights into decision-making processes at the individual level. As an expert guiding students through their econometrics assignments, my focus is on fostering a nuanced understanding of these models, equipping them with the skills to navigate the intricacies of discrete choice analysis. By mastering these models, students not only enhance their academic prowess but also prepare themselves for contributing valuable insights to real-world decision-making scenarios across diverse industries.
Deciphering Preferences: Applications and Interpretations of Discrete Choice Models
In the intricate realm of econometrics, the utilization of discrete choice models stands as a formidable tool for unraveling individual preferences. As an expert guiding students through the labyrinth of assignments, deciphering preferences through applications of these models is paramount. Discrete choice models, including multinomial logit and probit models, have become indispensable in understanding decision-making processes where individuals select among distinct alternatives.
The applications of these models span diverse fields such as transportation, marketing, and public policy. They enable economists to scrutinize consumer choices, electoral decisions, and market behaviors with a granularity that traditional models often lack. Through econometric assignments, students gain hands-on experience in crafting and interpreting these models, enhancing their analytical prowess and decision-making acumen.
The interpretation of results becomes a nuanced dance between statistical significance and economic intuition. A seasoned guide ensures students navigate this dance with finesse, emphasizing the importance of robustness checks and validating assumptions. The expert perspective inculcates an understanding of model limitations and the significance of contextualizing findings within real-world scenarios.
In essence, the applications and interpretations of discrete choice models in econometrics assignments not only sharpen students' analytical skills but also cultivate a deeper appreciation for the nuanced interplay between statistical methods and economic phenomena. The expert's role transcends mere guidance; it is a mentorship that equips future economists to unravel the intricacies of human choice in an ever-evolving world.
Conclusion:
In conclusion, the realm of microeconometric techniques serves as a crucial toolkit for understanding and dissecting individual and firm behavior within the intricate fabric of economic systems. As an expert guiding students through their Econometrics assignments, it is evident that the application of these techniques empowers aspiring economists to unravel the nuances of decision-making processes at the micro level.
The meticulous analysis of individual and firm behavior through microeconometric tools not only provides insights into economic agents' choices but also facilitates the identification of causal relationships and the estimation of key parameters. From linear regression models to more advanced methodologies such as instrumental variable approaches and panel data analysis, students are equipped with a diverse set of tools to address complex economic questions.
Moreover, the intersection of microeconometrics with contemporary issues, such as labor market dynamics, consumer behavior, and industrial organization, underscores its relevance in informing policy decisions and business strategies. The ability to discern patterns, causality, and heterogeneity in individual and firm responses to economic stimuli becomes a valuable skill set for students venturing into the field of Econometrics.
As students navigate through their assignments under the guidance of an expert, emphasis should be placed on mastering the practical application of these techniques. The integration of real-world datasets, hands-on experience with statistical software, and a nuanced understanding of econometric assumptions and limitations are integral components of fostering a comprehensive understanding of microeconometric methods.
In essence, the study of microeconometric techniques goes beyond the confines of traditional econometric exercises. It is a gateway to unraveling the complexities inherent in individual and firm decision-making, providing a foundation for evidence-based economic analysis. As students grapple with assignments in this field, they embark on a journey towards becoming adept practitioners capable of contributing meaningfully to the ongoing dialogue in economic research and policymaking.