- Unraveling the Basics of Spatial Econometrics: An In-Depth Exploration of Spatial Relationships in Economic Data
- Practical Applications of Spatial Econometrics: Transformative Insights for Regional Policy Analysis and Urban Economics
- Challenges and Future Directions in Spatial Econometrics: Addressing Computational Complexities and Emerging Trends
- Computational Complexities:
- Addressing Computational Challenges:
- Emerging Trends:
- Guiding Students Through Spatial Econometrics Assignments: Fostering Mastery and Ethical Application of Spatial Models
- Conclusion:
Spatial econometrics plays a pivotal role in unraveling the intricacies of economic phenomena, offering a unique lens through which to examine geographic and regional patterns. As an expert guiding students through their college assignments in econometrics, I emphasize the significance of comprehending spatial relationships in economic models. In this realm, we delve into spatial autocorrelation, capturing how economic variables are interrelated across different locations. If you need assistance with your econometrics assignment, don't hesitate to reach out for expert support.
When tackling assignments, students are encouraged to grasp the spatial heterogeneity present in regional data, recognizing that economic conditions vary geographically. Models incorporating spatial lag and spatial error terms are introduced to elucidate the interdependence among neighboring regions, contributing to a more nuanced understanding of economic dynamics.
Furthermore, my guidance underscores the integration of geographic information systems (GIS) with econometric techniques, facilitating a seamless fusion of spatial data and quantitative analysis. This multidisciplinary approach equips students with a holistic toolkit for dissecting regional economic patterns.
Navigating through the complexities of spatial econometrics empowers students to produce assignments that not only meet academic standards but also contribute to the broader discourse on regional economic disparities. As an expert mentor, I advocate for a synthesis of theoretical understanding and practical application, fostering a generation of economists adept at unraveling the spatial dimensions of economic challenges.
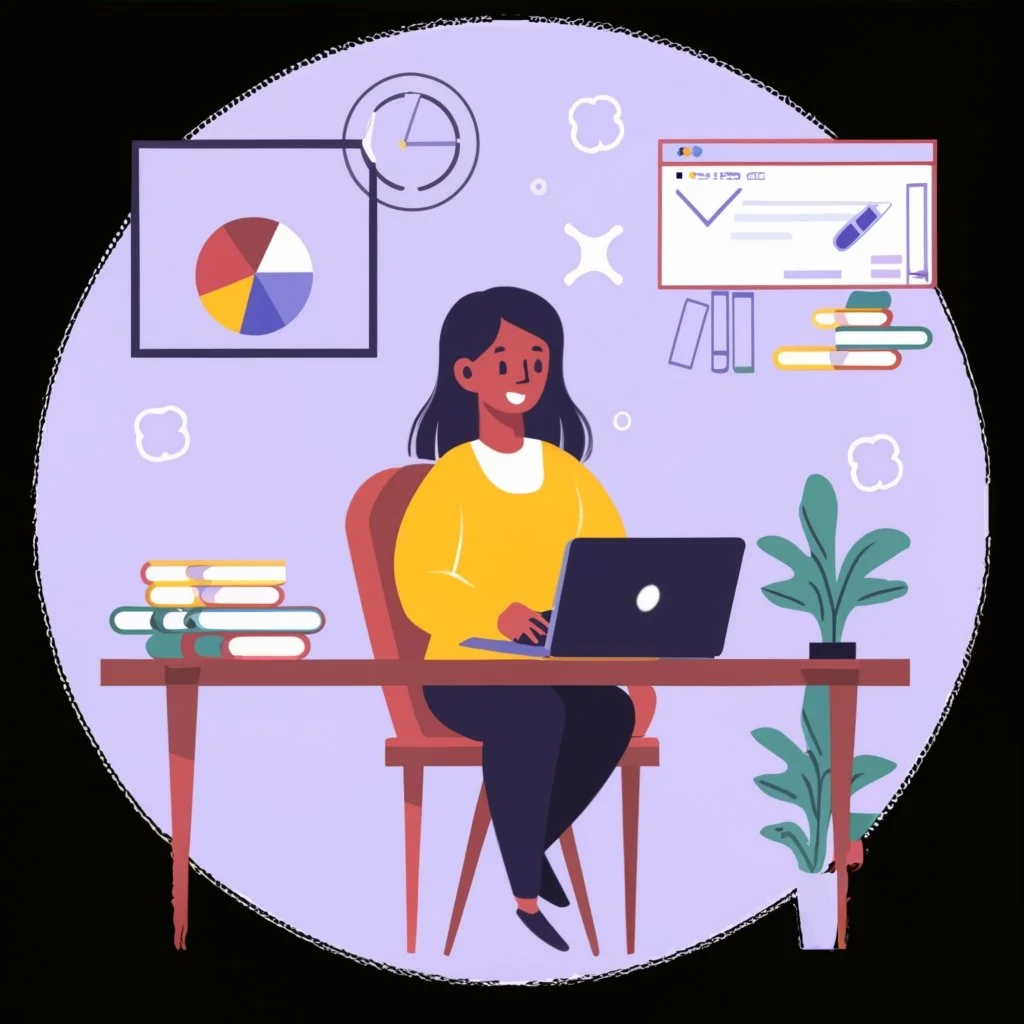
Unraveling the Basics of Spatial Econometrics: An In-Depth Exploration of Spatial Relationships in Economic Data
As an expert guiding students through the intricate landscape of econometrics, I find that one of the most captivating yet challenging realms lies in the application of spatial econometrics. This discipline delves into the spatial relationships inherent in economic data, offering a nuanced understanding of how geographical proximity and spatial dependencies can significantly impact economic phenomena. In this exploration, we will unravel the basics of spatial econometrics, shedding light on its principles and applications, serving as a beacon for students navigating this complex terrain in their assignments.
At its core, spatial econometrics extends traditional econometric methods by acknowledging that economic phenomena are not isolated entities but interconnected by geographical space. It recognizes that observations in close proximity may exhibit similar characteristics or share common influences. To capture and model these spatial dependencies, a specialized set of tools and techniques is employed, distinguishing spatial econometrics from its conventional counterpart.
Understanding the spatial autocorrelation is pivotal in comprehending the essence of spatial econometrics. This phenomenon denotes the tendency of neighboring observations to exhibit similarities, introducing a spatial structure that cannot be ignored in empirical analyses. Students embarking on spatial econometrics assignments must grasp the significance of spatial autocorrelation in shaping economic patterns, as it fundamentally alters the assumptions of classical econometric models.
Spatial econometrics introduces spatial weight matrices as a fundamental concept. These matrices define the strength and nature of the relationships between spatial units, reflecting the spatial structure of the data. Various forms of spatial weight matrices, such as contiguity-based or distance-based weights, offer students a diverse toolkit to capture the spatial interdependencies inherent in economic datasets. Mastery of these matrices is crucial for students seeking to unveil the spatial dynamics within their assigned projects.
Lurking beneath the surface of spatial econometrics is the Moran's I statistic, a metric that quantifies the degree of spatial autocorrelation. This statistical tool acts as a compass, guiding students in navigating the spatial landscape of their data. By interpreting Moran's I, students can discern whether their dataset exhibits clustering, dispersion, or randomness, providing valuable insights into the spatial patterns embedded in economic phenomena.
As students dive into the intricacies of spatial econometrics, they encounter spatial lag models and spatial error models, two prominent frameworks for modeling spatial dependencies. The former considers the influence of neighboring observations on the dependent variable itself, while the latter accounts for the spatial structure in the error term. Choosing between these models demands a careful consideration of the underlying spatial processes at play, and students must grapple with the implications of each model for their specific econometric assignment.
Moreover, spatial econometrics extends its reach to spatial heterogeneity, recognizing that spatial units may possess distinct characteristics. This facet introduces the concept of spatial regimes, prompting students to explore how economic relationships vary across different spatial contexts. By accommodating spatial heterogeneity, students can refine their econometric analyses to capture the nuances of diverse spatial subgroups within their datasets.
The realm of spatial econometrics unveils a captivating dimension of economic analysis, requiring students to navigate the intricacies of spatial relationships in their assignments. As an expert guiding these eager minds, I emphasize the importance of mastering spatial autocorrelation, spatial weight matrices, Moran's I, and the choice between spatial lag and error models. By delving into these foundational concepts, students can unlock the potential to unravel the spatial dynamics inherent in economic data, elevating their econometric analyses to a new level of sophistication and insight.
Practical Applications of Spatial Econometrics: Transformative Insights for Regional Policy Analysis and Urban Economics
Spatial econometrics, a subfield of econometrics, has emerged as a powerful tool for understanding the complex relationships that exist within regional economies and urban landscapes. As an expert guiding students through their econometrics assignments, I find myself consistently impressed by the transformative insights that spatial econometrics provides for regional policy analysis and urban economics. This methodology goes beyond traditional econometric techniques by explicitly considering the spatial dependencies and interactions that exist in economic data, offering a nuanced understanding of how location and proximity impact economic phenomena.
One of the practical applications of spatial econometrics lies in regional policy analysis. Governments and policymakers grapple with the challenge of formulating effective policies that can address regional disparities and promote balanced development. Spatial econometrics allows us to model the spatial interdependencies among regions, providing a more accurate representation of the dynamics at play. For example, when assessing the impact of an infrastructure project on regional development, spatial econometrics can account for the fact that neighboring regions may experience spillover effects. This ensures that policymakers can make informed decisions based on a comprehensive understanding of how interventions in one area may reverberate throughout the entire region.
Urban economics, another domain greatly enriched by spatial econometrics, benefits from a more nuanced understanding of spatial interactions within cities. Urban areas are complex systems with intricate relationships between different neighborhoods and districts. By incorporating spatial considerations into econometric models, researchers and policymakers can gain insights into issues such as housing prices, transportation networks, and the spatial distribution of economic activities. For instance, when analyzing housing markets, spatial econometrics helps account for the fact that the value of a property is not only influenced by its inherent characteristics but also by the characteristics of neighboring properties and the overall neighborhood.
Furthermore, spatial econometrics aids in tackling the challenges posed by spatial heterogeneity, where different regions or locations exhibit distinct characteristics. This is crucial in regional studies where variations in terrain, climate, or cultural factors can significantly impact economic outcomes. Students engaging with spatial econometrics in their assignments learn to employ techniques like spatial autoregressive models, spatial lag models, and spatial error models to address these challenges. These models enable them to discern the spatial patterns of economic phenomena, providing a more accurate picture of the underlying dynamics.
In the realm of practical applications, students also explore the role of spatial econometrics in addressing spatial autocorrelation, a phenomenon where observations in close proximity tend to have similar values. This is particularly relevant in urban economics, where spatial autocorrelation can manifest in various forms, such as the clustering of similar businesses in certain neighborhoods. Spatial econometrics equips students with tools like Moran's I statistic and spatial weight matrices to detect and correct for spatial autocorrelation, ensuring that their analyses are robust and reliable.
The practical applications of spatial econometrics are instrumental in shaping regional policy analysis and urban economics. As an expert guiding students through their econometrics assignments, I emphasize the importance of incorporating spatial considerations into their analyses. By doing so, they not only enhance the accuracy and reliability of their models but also contribute to a deeper understanding of the intricate spatial relationships that underlie economic phenomena. In an increasingly interconnected and dynamic world, spatial econometrics stands as a transformative tool, empowering students to navigate the complexities of regional and urban economic analysis with precision and insight.
Challenges and Future Directions in Spatial Econometrics: Addressing Computational Complexities and Emerging Trends
As an expert guiding students through the intricate realm of spatial econometrics, it is imperative to acknowledge the evolving landscape of this discipline. Spatial econometrics, a subfield of econometrics, has witnessed remarkable advancements over the years, presenting both challenges and exciting prospects for scholars and researchers. In this discourse, we delve into the complexities faced by students and practitioners in spatial econometrics, emphasizing the imperative need to address computational challenges while also exploring emerging trends that shape the future of this dynamic field.
Computational Complexities:
Spatial econometrics deals with the intricacies of spatial dependencies and interactions among economic variables. However, the increasing complexity of models and datasets poses a significant challenge for students navigating this field. One of the primary computational hurdles lies in the estimation of spatial econometric models, especially when dealing with large datasets. The sheer volume of spatial data necessitates sophisticated algorithms and computing power, pushing the boundaries of conventional statistical software.
The spatial econometric models, such as spatial autoregressive models and spatial error models, often require intricate matrix manipulations and spatial weight matrix calculations. As students strive to implement these models, they encounter challenges related to the computational intensity and the demand for high-performance computing resources. This prompts the need for expertise in programming languages and tools like Python, R, or specialized software packages tailored for spatial econometrics.
Moreover, the integration of spatial data into econometric analyses introduces challenges in terms of data preprocessing and the construction of appropriate spatial weight matrices. Students must grapple with issues such as spatial autocorrelation, heteroscedasticity, and endogeneity, demanding a nuanced understanding of econometric theory and advanced statistical techniques.
Addressing Computational Challenges:
To overcome these challenges, students must hone their programming skills and acquaint themselves with computational tools specifically designed for spatial econometrics. Investing time in learning Python or R and mastering spatial data manipulation libraries becomes essential. Moreover, the utilization of parallel computing and distributed computing frameworks can significantly enhance the efficiency of computations, enabling the estimation of complex spatial econometric models within reasonable timeframes.
Furthermore, collaboration with experts in computer science and data science is encouraged. As an expert guiding students, I emphasize the importance of interdisciplinary collaboration. Working with experts in computational methods can provide students with valuable insights and solutions to address the computational challenges posed by spatial econometrics.
Emerging Trends:
Looking towards the future, spatial econometrics is poised to embrace emerging trends that will shape the discipline. The integration of machine learning techniques, such as deep learning and spatial neural networks, presents exciting avenues for research and analysis. These techniques have the potential to capture complex spatial patterns and relationships in data, pushing the boundaries of traditional spatial econometric methods.
Additionally, the growing availability of high-resolution spatial data from diverse sources, including satellite imagery and geospatial sensors, opens up new possibilities for spatial econometric analyses. Students should be encouraged to explore these rich datasets and leverage cutting-edge technologies to enhance the precision and scope of their spatial econometric studies.
As an expert guiding students in spatial econometrics, it is crucial to address the computational challenges posed by the discipline while also nurturing an awareness of emerging trends. By equipping students with the tools to navigate computational complexities and encouraging exploration of innovative methodologies, we ensure that the future of spatial econometrics remains vibrant and at the forefront of empirical research in economics.
Guiding Students Through Spatial Econometrics Assignments: Fostering Mastery and Ethical Application of Spatial Models
As an expert in the field of econometrics, my primary goal is to guide students through the intricate landscape of spatial econometrics assignments, facilitating not only the mastery of complex models but also instilling a sense of ethical responsibility in their application. The realm of spatial econometrics delves into the spatial dependencies and interactions that exist in economic phenomena, requiring students to navigate a terrain where statistical methodologies intersect with geography, offering both challenges and opportunities for deeper insights.
The journey begins by establishing a solid foundation in traditional econometric principles. Understanding the basics of regression analysis, hypothesis testing, and model specification is essential before delving into the spatial dimension. A well-structured curriculum ensures that students comprehend the nuances of econometric models, setting the stage for a seamless transition to spatial econometrics.
Spatial econometrics introduces a paradigm shift by acknowledging the spatial interdependence of observations. This necessitates a departure from the assumption of independence that underlies classical econometrics. Students are guided to explore the spatial autocorrelation and heterogeneity inherent in their datasets, emphasizing the need for specialized tools and models to account for these spatial dependencies.
In guiding students, I emphasize the ethical implications of spatial econometric applications. Recognizing the potential for biased results and misleading interpretations, students are encouraged to approach their assignments with a keen awareness of the broader implications of their analyses. The ethical use of spatial models demands transparency, rigorous validation of assumptions, and a commitment to reporting unbiased results.
One key aspect of guiding students through spatial econometrics assignments is fostering critical thinking. Students are encouraged to question assumptions, consider alternative models, and assess the robustness of their findings. This not only enhances their analytical skills but also instills a sense of intellectual curiosity that is essential in a field that constantly evolves with emerging methodologies and data sources.
Practical application is another crucial component of the learning process. Real-world datasets are employed to bridge the gap between theoretical concepts and their implementation. Students engage in hands-on exercises, applying spatial models to address relevant economic questions. This experiential learning approach enhances their ability to translate theoretical knowledge into actionable insights, preparing them for the challenges they may encounter in their professional endeavors.
In guiding students through spatial econometrics assignments, I emphasize the importance of effective communication. The ability to convey complex findings in a clear and accessible manner is a skill that transcends academic settings. Students are coached on how to present their results, interpret spatial patterns, and communicate the implications of their analyses to diverse audiences, fostering a well-rounded skill set that extends beyond the technical aspects of econometrics.
Furthermore, ethical considerations extend to the responsible use of spatial data. Students are educated on issues related to data privacy, confidentiality, and the potential for spatial models to perpetuate biases if not applied thoughtfully. By fostering a commitment to ethical conduct, students are better equipped to contribute positively to the field, using spatial econometrics as a tool for informed decision-making rather than a means to an end.
In conclusion, guiding students through spatial econometrics assignments is a multifaceted endeavor that goes beyond imparting technical skills. It involves cultivating a deep understanding of the ethical dimensions of spatial modeling, fostering critical thinking, and honing practical application skills. By empowering students in these areas, we not only contribute to their academic success but also prepare them to navigate the complex intersection of econometrics and spatial analysis in an ethically responsible manner.
Conclusion:
In conclusion, delving into the realm of spatial econometrics provides students with a powerful toolkit to analyze economic phenomena in a geographically nuanced manner. As an expert guiding students through their econometrics assignments, it is evident that understanding geographic and regional economic models is essential for comprehending the spatial dimensions of economic relationships. The incorporation of spatial elements into econometric analyses allows for a more realistic depiction of economic interactions, acknowledging the influence of proximity and spatial dependencies.
Through this exploration, students gain a deeper insight into the complexities of regional economies, uncovering patterns and interdependencies that traditional econometric models may overlook. Spatial econometrics equips them with the means to discern and quantify spatial spillover effects, thereby enhancing the accuracy and applicability of economic analyses.
Furthermore, the adoption of spatial econometrics in college assignments fosters a bridge between theoretical concepts and practical applications. Students not only grasp the theoretical underpinnings of economic models but also develop the skills to implement them in real-world scenarios. This not only enhances their academic prowess but also prepares them for the challenges they may encounter in their future careers.
The interdisciplinary nature of spatial econometrics encourages students to draw connections between economics, geography, and statistics, fostering a holistic understanding of economic phenomena. As an expert guide, it is rewarding to witness students navigate the complexities of spatial econometrics, as they acquire the analytical acumen necessary to contribute meaningfully to the field.
In conclusion, spatial econometrics stands as a dynamic and evolving field that empowers students to explore the intricate relationships between space and economics. As they engage with geographic and regional economic models, students not only hone their analytical skills but also contribute to advancing our comprehension of spatially influenced economic processes. The incorporation of spatial econometrics into college assignments not only enriches the learning experience but also equips students with a valuable set of tools to navigate the intricacies of economic analysis in an ever-changing global landscape.